By Chainika Thakar and Anupriya Gupta
Unleashing a technological revolution and reshaping the stock market landscape, algorithmic trading, high-frequency trading (HFT), and news-based trading have become the key players.
Now, let me ask you certain questions.
How have computer programs evolved into powerful allies, automating trading decisions?
What secrets lie behind the lightning-fast execution of HFT, seizing minuscule market chances?
How has news based trading become so impactful in the trading domain and what can you do to utilise news based information for your trading practice?
You can find out all about it in this blog.
Explore the realm of news-based trading, where real-time updates become the catalyst for informed choices. Witness the evolution of stock market regulations, guarding the sacred principles of fairness and transparency amidst this thrilling era of transformation. Brace yourself for a captivating journey into the captivating history of algorithmic trading.
Let us find out all about the history of algorithmic trading, HFT and news based trading with this blog that covers:
- Algorithmic trading, HFT and News based trading
- History of algorithmic trading, HFT and news based trading
- Future outlook for algorithmic trading, HFT and News based trading
- The rules and regulations of stock market over a period of time
- The top firms involved in algorithmic trading and HFT
Algorithmic trading, HFT and News based trading
History of algorithmic trading, high-frequency trading (HFT), and news-based trading is quite interesting. All the three approaches are used in financial markets to execute trades. While they share this similarity of belonging to the trading domain, they also have distinct differences.
First of all, let us see an explanation of each below:
Algorithmic trading
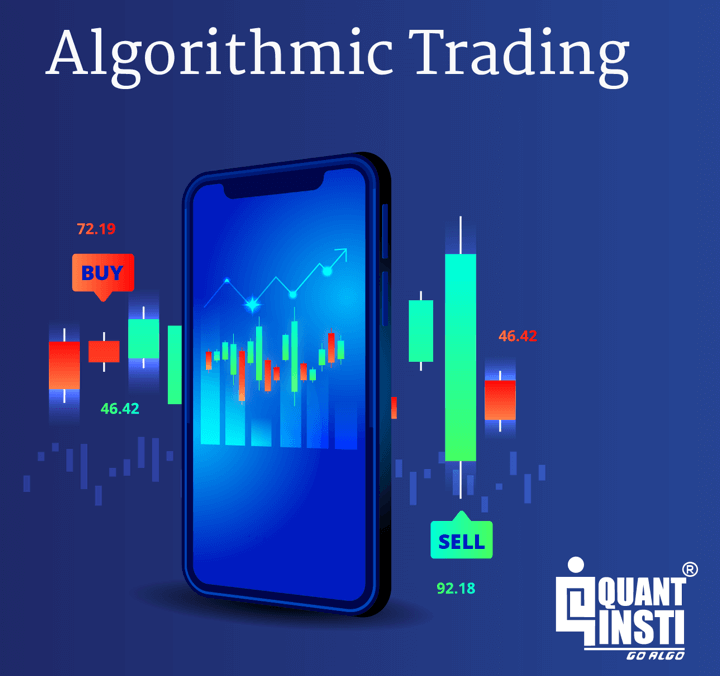
Example:
An example of algorithmic trading could be a quantitative hedge fund that uses complex mathematical models to identify patterns in historical price data. The algorithm may be programmed to generate buy or sell signals based on specific technical indicators, such as moving averages or volatility levels. The trades are executed automatically by the system, aiming to capture short-term price movements and exploit market inefficiencies.
For those delving into algo trading for beginners, understanding such examples highlights the importance of integrating data analysis and automation. It provides a clear picture of how algorithms can simplify decision-making and enhance trading efficiency.
Both HFT and news-based trading can also utilise algorithmic techniques with the help of computer languages such as Python, C etc. The automation and the predefined rules can be given to the system to exploit the market opportunities as and when they arise.
High-Frequency Trading (HFT)
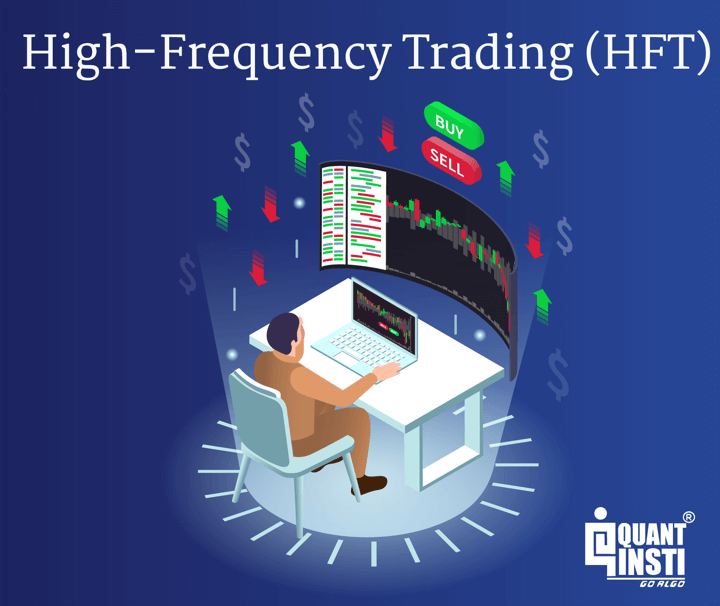
Example:
An example of HFT could be a proprietary trading firm that uses advanced algorithms and high-speed connections to execute trades on multiple exchanges. The algorithms analyse real-time market data, such as order book changes and price movements, to identify fleeting trading opportunities. The HFT system quickly sends out orders and captures small price discrepancies, aiming to profit from these fast-paced trades.
Both algo trading and news-based trading (news trading strategies) can incorporate HFT techniques to capitalise on market inefficiencies and exploit small price discrepancies.
News-Based Trading
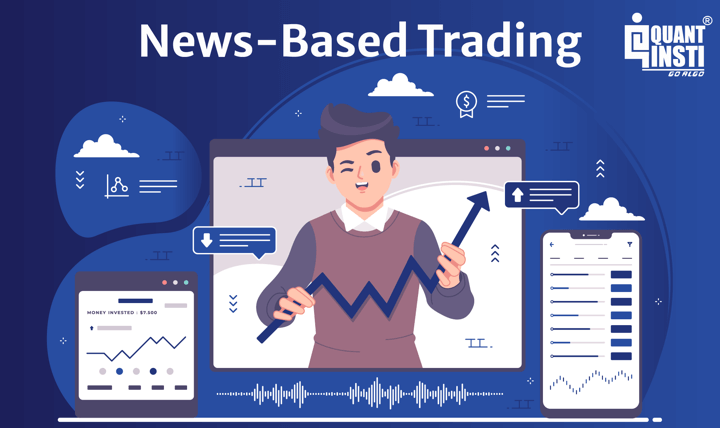
Example:
An example of news-based trading could be a hedge fund that uses algorithms to monitor news feeds and social media platforms for real-time updates on companies and industries. The algorithms analyse the sentiment and relevance of the news articles and generate trading.
News-based trading can incorporate algorithmic techniques for data analysis and trade execution. Both algorithmic trading and HFT can incorporate news-based strategies. Expand your expertise by diving into Algorithmic Trading books.
Key Differences
Let us now see what distinguishes each of the trading types from the other and makes each unique.
Topic |
Algorithmic trading |
HFT |
News based trading |
Objectives |
Algorithmic trading aims to optimise trade execution, improve efficiency, and capitalise on market opportunities. |
HFT focuses on high volumes of trades and exploiting small price discrepancies. |
News-based trading aims to capture market movements driven by news events and announcements. |
Time horizon |
Algorithmic trading can encompass various time horizons, from short-term to long-term. |
HFT specifically focuses on ultra-fast trade execution within microseconds. |
News-based trading can have varying time horizons depending on the impact and duration of the news event. |
Speed and frequency |
Algorithmic trading aims to optimise trade execution based on predefined rules, but it may not require the same level of ultra-high speed as HFT. |
HFT strategies, on the other hand, heavily rely on speed and frequency to capture fleeting opportunities. |
News-based trading requires quick analysis and response to news events but may not necessarily require the same level of speed as HFT. |
Data sources |
Algorithmic trading can utilise various data sources, including historical price data, technical indicators, and quantitative models. |
HFT often focuses on real-time market data and order book information. |
News-based trading relies on news sources, such as news feeds and social media, and utilises NLP techniques to extract relevant information and sentiment from the news |
History of algorithmic trading, HFT, and news based trading
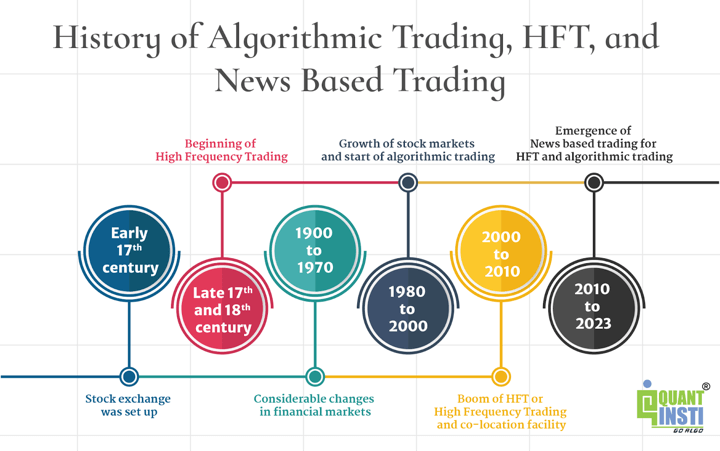
Early 17th century: Stock exchange was set up
The 17th century saw the emergence of the Dutch East India Company (VOC), which revolutionised trading by introducing transferable shares and creating a secondary market in Amsterdam. This laid the foundation for the city's transformation into a major financial hub. The introduction of tradable shares led to increased trading activity and the development of sophisticated techniques like forwards, futures, options, and bear raids. By 1680, the trading practices in Amsterdam closely resembled those seen in modern financial markets, showcasing the city's early role as an innovator and setting the stage for advanced techniques worldwide.
The 17th century marked a turning point in trading history with the VOC's introduction of transferable shares. Amsterdam's market flourished, incorporating complex transactions and becoming a prominent financial centre. This period's innovations played a crucial role in shaping global trading, paving the way for advanced techniques and the growth of financial centres worldwide.
Late 17th and 18th century: Beginning of High Frequency Trading
High-Frequency Trading (HFT) revolves around the speed of information transmission. HFT traders employ cutting-edge technology to obtain information faster than their counterparts and execute trades swiftly. Interestingly, the concept of rapid information delivery has roots that trace back to the 17th century.
The establishment of the London Stock Exchange in the late 18th century created a centralised marketplace for securities trading, setting the stage for the development of High-Frequency Trading (HFT). The exchange's technological advancements and infrastructure made it conducive for HFT firms to operate, taking advantage of its speed and efficiency in executing trades.
1900 to 1970: Considerable changes in financial markets
From 1900 to 1929, railways faced challenges, such as changing transportation technologies, competition from automobiles and planes, and economic recessions, leading to their decline. Meanwhile, the financial sector rose in importance. These shifts reflected broader economic and societal changes that affected the market and investor sentiment.
In the early 1970s, computerization of order flow in financial markets began. The New York Stock Exchange introduced systems like (Designated Order Turnaround) DOT and later SuperDOT, which electronically routed orders for manual execution.
On the other hand, Julius Reuter, the founder of Thomson Reuters, revolutionised information dissemination in the 19th century. His innovative news delivery system utilised telegraph cables and carrier pigeons for swift communication, laying the foundation for high-frequency trading.
1980 to 2000: Growth of stock markets and start of algorithmic trading
A significant structural change occurred from around 1982 to 2007 when the financial sector experienced remarkable growth and influence in the U.S. stock market. During this period, factors such as financial regulation, technological advancements, and innovation in financial products contributed to the rise of the financial sector.
Institutions such as investment banks, commercial banks, and other financial intermediaries became increasingly prominent, driving the market's performance and shaping its dynamics.
You must be wondering as to when did algorithmic trading start?
Algorithmic trading emerged with the advent of the internet in the late 1980s and early 1990s.
In the late 1980s and 1990s, financial markets transitioned to electronic execution and the development of electronic communication networks (ECNs). Decimalisation in the US reduced bid-ask spreads, encouraging algorithmic trading and increasing market liquidity.
The authorisation of electronic exchanges by the SEC in 1998 paved the way for high-frequency trading (HFT). HFT utilises advanced technology to execute trades at speeds thousands of times faster than humans, leading to its widespread adoption and significant impact on financial markets.
2000 to 2010: Boom of HFT or High Frequency Trading and co-location facility
In the early 2000s, high-frequency trading accounted for less than 10% of equity orders. However, it quickly gained traction and grew rapidly over the years.
According to the NYSE, between 2005 and 2009, high-frequency trading volume experienced a remarkable 164% increase.
2011, marked the year of launching Nano trading technology. A firm called Fixnetix developed a microchip that could execute trades in nanoseconds, which is equal to one billionth of a second.
Hence,
1 Nanosecond = 0.000000001 seconds
Co-location implies locating computers owned by HFT firms and proprietary traders in the same premises where an exchange’s computer servers are housed.
Co-location began gaining prominence in the early 2000s. It was during this time that exchanges started offering co-location services,
In the quest for speed Denver-based data centre company CoreSite, which operates a facility where traders can install so-called "co-located" computers right in the heart of Washington.
To deepen your understanding, you can watch a video on Trading in Milliseconds that explores the topics and setups discussed above. (Check out the course on Millisecond trading by Quantra)
Trading in Milliseconds: MFT Strategies and Setup | An Introduction to the Course | Quantra
2010 to 2023: Emergence of News based trading for HFT and algorithmic trading
The advent of the internet in the late 20th century had a profound impact on news-based trading. It enabled the instantaneous dissemination of news globally, levelling the playing field for traders. Online news platforms, financial websites, and social media channels became primary sources of news for investors, offering real-time information and analysis.
In September 2012, Dataminr launched a service that turned social media streams into actionable trading signals, backed by a $30 million investment. It provided clients with business news up to 54 minutes faster than traditional sources.
The platform identified micro-trends by analysing on-the-ground chatter, consumer product reactions, online community discussions, and public attention patterns. Dataminr's innovation leveraged social media data for real-time insights, reflecting the increasing role of AI and machine learning in finance. Take control with a feature-rich Algorithmic Trading Platform.
Artificial intelligence in trading has become pivotal for real-time data processing and strategic analysis. By using AI, platforms can interpret market sentiments and respond to emerging patterns faster than traditional methods, enhancing the effectiveness of trading decisions and risk management.
In 2012, real-time analytics engines analysed the vast number of daily tweets to identify linguistic and propagation patterns. During that time, High-Frequency Trading (HFT) dominated the stock markets, accounting for 70% of US equity trades.
Over the past few years, trading precision has increased, with stock prices moving from fractions to pennies. High-frequency trading (HFT) has added liquidity to the market and reduced bid-ask spreads.
IT companies have invested heavily in HFT technology, including ultra-fast computer chips that execute trades in microseconds. Additionally, a proposed $300 million transatlantic cable aimed to reduce transaction times between New York City and London by a fraction of a second.
The monitoring of social media by the FBI began and this has led to the instant impact of the social media on the securities. On April 2nd 2013 the SEC and CFTC levied restrictions on public company announcements through social media.
Twitter data for trading
Just two days after the restrictions by the SEC and CFTC on April 4th 2015, Bloomberg Terminals incorporated live Tweets into its economic data service. Bloomberg Social Velocity tracked abnormal spikes in chatter about specific companies.
A noteworthy example of an abnormal news item affecting stock markets was on November 11, 2022 – the day a false Tweet sent for Eli Lilly and Company by an impostor. Eli Lilly’s stock tumbled more than 5% on Friday and was still down more than 4% at the close. The company lost over $15 billion in market cap as a result of the mischievous tweet.
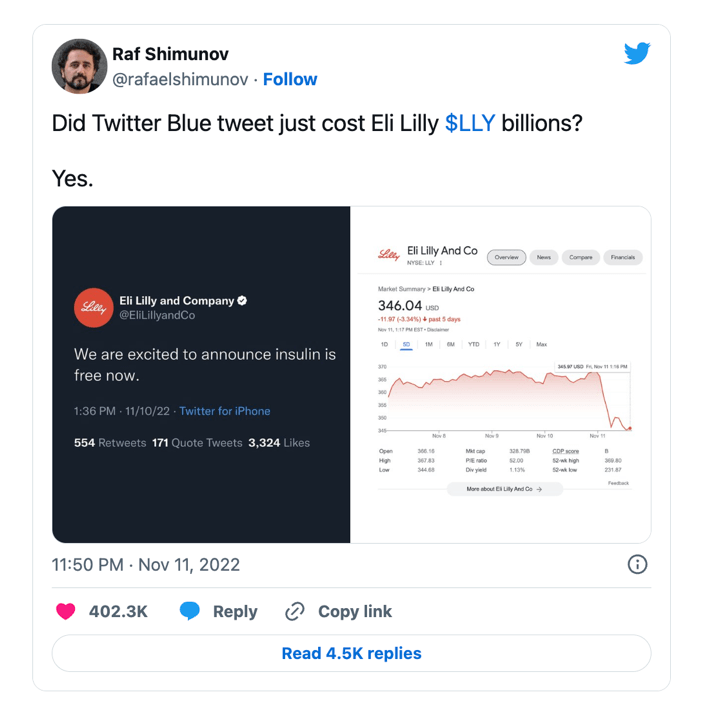
With natural language processing (NLP) and machine learning, traders can process and analyse large volumes of real-time textual data. By incorporating news-based signals into their decisions, they gain insights, anticipate market reactions, and identify trends. However, challenges include the need for fast data processing, accurate interpretation of news, and distinguishing significant events from noise.
However, it is important to note that news-based trading also comes with challenges. The speed at which news travels and the need for rapid data processing and analysis require sophisticated technological infrastructure and algorithms. Additionally, the interpretation of news and the ability to differentiate between noise and significant events can be a complex task.
Future outlook for algorithmic trading, HFT and News based trading
The future outlook for algorithmic trading, High-Frequency Trading (HFT), and News-based trading is highly promising.
News-based trading continues to gain popularity as traders seek alternative data sources and innovative strategies to gain an edge in the markets. The integration of news analysis with algorithmic trading and other quantitative techniques is likely to further shape the landscape of news-based trading in the future.
The following significant advancements and developments are expected from each:
Algorithmic Trading
- Increased sophistication and refinement of algorithms
- Faster and more accurate decision-making capabilities
- Optimization of execution strategies
- Enhanced ability to identify and exploit profitable opportunities
- Integration of advanced technologies such as AI and machine learning
High-Frequency Trading (HFT)
- Continued investment in cutting-edge infrastructure and technology
- Exploitation of microsecond-level market inefficiencies
- Adaptation to evolving regulatory frameworks
- Focus on maintaining fairness, transparency, and market integrity
- Emphasis on risk management and system resilience
News-based Trading
- Advancements in natural language processing and sentiment analysis
- Faster access to real-time news and information
- Improved prediction of market reactions to news events
- Enhanced understanding of market sentiment and trends
- Integration of news analytics into trading strategies
Note: It's important to note that these expectations are subject to technological advancements, market conditions, and regulatory developments. Traders and market participants should stay informed and adapt to the evolving landscape to leverage the full potential of each type of trading.
The rules and regulations of stock market over a period of time
Here are some key historical milestones of rules and regulations for stock market trading:
Securities Act of 1933 and Securities Exchange Act of 1934 (United States)
These acts were enacted to regulate the issuance, sale, and trading of securities, and establish the U.S. Securities and Exchange Commission (SEC) to oversee the securities industry.
Securities Investor Protection Act (SIPA) of 1970 (United States): This act created the Securities Investor Protection Corporation (SIPC), which provides limited protection to investors in case of broker-dealer failures.
The Big Bang (1986) in the United Kingdom
This event marked the deregulation of the London Stock Exchange, allowing electronic trading, increased competition, and foreign participation in the market.
Regulation ATS (Alternative Trading Systems) in 1998 (United States): This regulation introduced a framework for the operation of electronic trading systems that match buy and sell orders outside traditional stock exchanges.
Regulation NMS (National Market System) in 2005 (United States)
This regulation aimed to promote fair competition, transparency, and efficient markets by requiring certain standards for trading, order execution, and access to market data.
Dodd-Frank Wall Street Reform and Consumer Protection Act in 2010 (United States): This legislation introduced significant regulatory reforms in response to the financial crisis of 2008, including measures to enhance market transparency, regulate derivatives trading, and strengthen oversight of financial institutions.
MiFID II (Markets in Financial Instruments Directive II) in 2018 (European Union)
This regulatory framework introduced stricter rules on transparency, investor protection, and market structure for financial markets within the European Union.
The top firms involved in algorithmic trading and HFT
Now let us find out some of the top most firms that are involved in algorithmic trading, HFT and news based trading.
Some of the Global HFTs and Algorithmic Trading firms
- Renaissance Technologies
- Jane Street Capital
- Citadel Securities
- Virtu Financial
- Tower Research Capital
- Jump Trading
- DRW
- Hudson River Trading
- Two Sigma Securities
- Flow Traders
- IMC Financial Markets
- XR Trading
Further reads
- Algorithmic trading with ChatGPT
- The Impact of High Frequency Trading on Institutional Investors
- Rise of the Machines: Algorithmic Trading in the Foreign Exchange Market
- Sentiment Analysis
- Trading Strategies to Exploit Blog and News Sentiment
Bibliography
- https://algomojo.com/blog/evolution-of-algorithmic-trading/
- The externalities of High Frequency Trading
- High Frequency Trading
- The causal impact of algorithmic trading on market quality
Conclusion
Algorithmic trading, HFT, and news-based trading have revolutionised the stock market landscape, driven by technological advancements and regulatory developments. These practices have enabled faster trade execution, increased liquidity, and provided unique insights from real-time news and data. The industry continues to evolve, with firms competing to stay at the forefront of technological innovation and trading strategies.
If you wish to learn more about algorithmic trading, HFT and news based trading, do explore our Algo Trading course.
If you are a professional looking to advance in the field of trading or considering a career in Algorithmic and Quantitative Trading?
Then, the Algorithmic Trading course is meant for you. This program is specifically designed to inspire traditional traders and equip them with the necessary skills in derivatives, quantitative trading, electronic market-making, trading technology, and risk management. Explore this course and enrol to pave the way for a successful algorithmic trading journey!
Note: The original post has been revamped on 21st August 2023 for accuracy, and recentness.
Disclaimer: All data and information provided in this article are for informational purposes only. QuantInsti® makes no representations as to accuracy, completeness, currentness, suitability, or validity of any information in this article and will not be liable for any errors, omissions, or delays in this information or any losses, injuries, or damages arising from its display or use. All information is provided on an as-is basis.