Machine learning is essential across diverse sectors such as medicine, transportation, healthcare, advertising, and financial technology. In the realm of financial technology, machine learning greatly enhances algorithmic trading efficiency.
During your quest to learn all about machine learning, have you ever wished for a one-stop hub with free resources like courses, blogs, e-books, research papers, and videos?
Well, I’m sure you must have! And, you've just found it! This is a tailored guide to help you with all the knowledge goodies. Whether you're into interactive courses, thought-provoking blogs, or deep dives into e-books, consider this blog your treasure map.
These resources are categorised for easy navigation.
Disclaimer: We are not associated with the mentioned publications and/or courses in any way (except for the ones from Quantra and/or QuantInsti). All the mentions are for the sheer purpose of learning.
So, let's make learning a breeze and turn that quest of yours for knowledge into an adventure. Get ready to explore the ultimate resource haven with this blog that covers:
Courses
First of all, let us see some interesting and useful courses which are completely free of cost.
- Introduction to Machine Learning for Trading
This free course is perfect for beginners in using machine learning for trading. The course helps you learn how the machine learning algorithms are implemented on financial markets data.
Learn to create robust predictive models for algo trading with this course. After completing this course, you will have in-depth knowledge of the concepts of machine learning for trading.
This is what the first page of the course looks like. Click on the “Login” on the top right and then click on “Enrol for Free”.
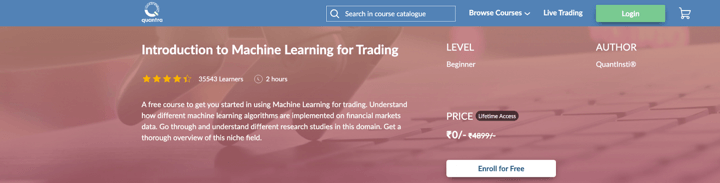
This course is a part of an 8-course Learning Track titled “8-course guide to quantitative trading for beginners”.
2. Machine learning in Python with scikit-learn
This course provides step-by-step lessons, offering fundamental skills for machine learning and serving as a foundation for advanced pursuits in AI, text mining, or data science.
Beyond being mere knowledge, it instils critical thinking in designing predictive models. Learn to scrutinise each step, from data preprocessing to model selection, understanding failure modes, and interpreting predictions.
Expect hands-on training, featuring practical applications with participant-executed code. course materials are accessible on a GitHub repository. Guided by scikit-learn core developers, this course ensures a robust learning experience.
With this free course, you will embark on a comprehensive journey into predictive modelling with scikit-learn. Scikit-learn is a popular Python library helping the coders with a wide array of tools for data analysis. Here is how the first page looks like:
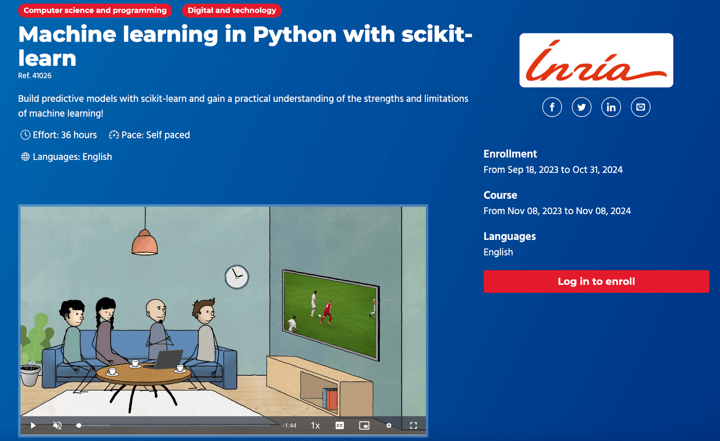
E-Books
- Machine learning in trading by Quantra
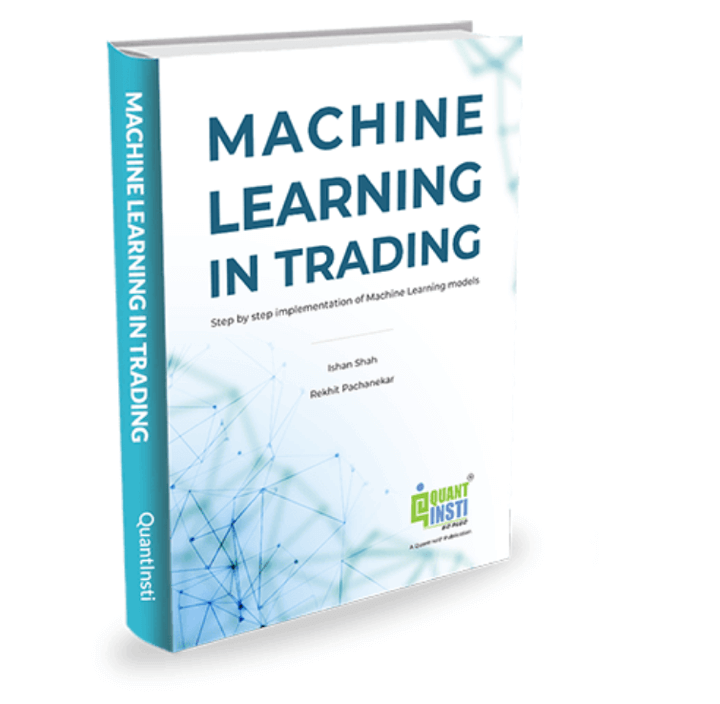
This eBook contains all the information, from explaining the basics and working of artificial neural networks, to demonstrating the code to implement it in Python for stock price prediction.
Aim of the book is to:
- Demystify machine learning concepts, including AI, Neural Networks, and Deep Learning.
- Clarify distinctions between machine learning and traditional programming.
- Help you explore practical applications of machine learning for gaining a trading advantage.
- Structure the learning approach which implies understanding the tasks first, then delving into corresponding Python code.
2. Interpretable Machine Learning, by Christoph Molnar
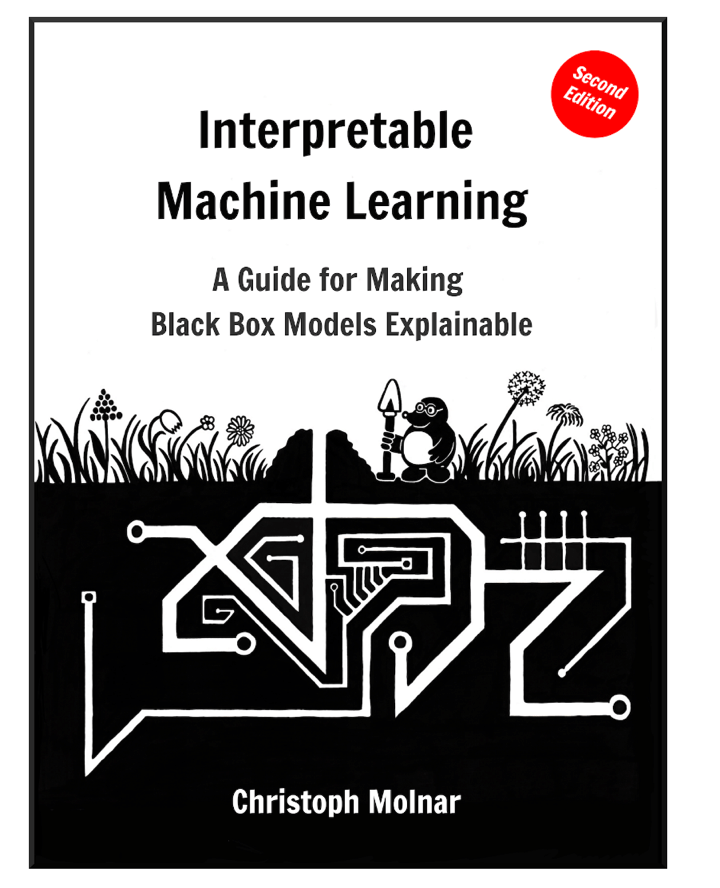
This book focuses on helping the readers learn the machine learning models for tabular data. To learn machine learning for trading, this book will help acquire the knowledge of relational or structured data which is one of the important concepts while trading using machine learning.
Aim of the book is to:
- Enable readers to grasp machine learning models tailored for tabular data.
- Foster understanding of relational or structured data crucial for trading with machine learning.
- Facilitate the acquisition of knowledge specific to applying machine learning in trading contexts.
3. Fighting Churn with Data, by Tien Tzuo, Founder and CEO, Zuora
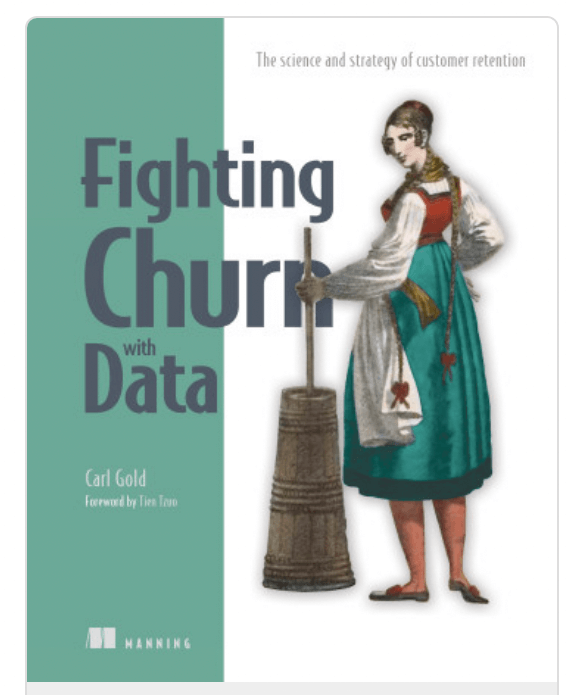
This machine learning book is meant for data analysts and data scientists who are an integral part of algorithmic trading. Chapter 9 of the book covers the concept of backtesting which is useful in algorithmic trading. This book also covers the XGBoost machine learning model and cross validation technique. Learn more with these must-read Algorithmic Trading books.
Aim of the book is to:
- Target the data analysts and data scientists involved in algorithmic trading.
- Put the emphasis on the pivotal role of backtesting in algorithmic trading.
- Comprehensively cover the XGBoost machine learning model.
- Lead to an in-depth exploration of the cross-validation technique.
4. Learning From Data, by Yaser S. Abu-Mostafa, Malik Magdon-Ismail, Hsuan-Tien Lin
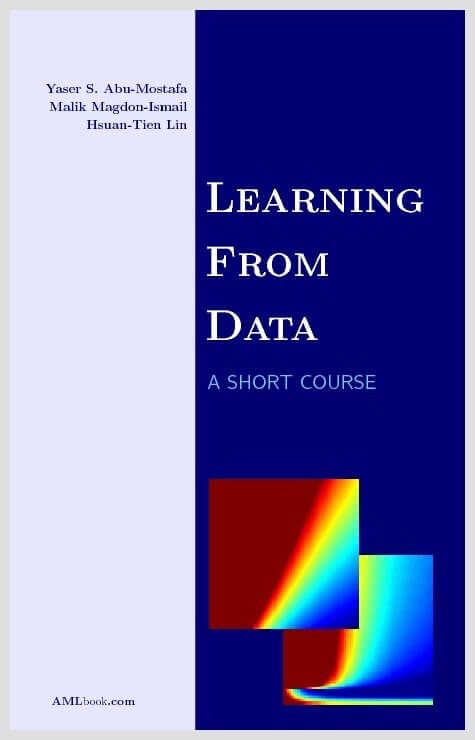
This book provides the reader with a complete introduction to machine learning, the technology that enables computational systems to adaptively improve their performance with experience accumulated from the observed data. Such techniques are widely applied in engineering, science, finance, and commerce. Chapter 5 of this book touches upon the application of machine learning in trading.
Aim of the book is to:
- Provide a thorough understanding of machine learning as a technology driving adaptive performance improvement in computational systems.
- Highlight the broad application of machine learning in engineering, science, finance, and commerce.
- Emphasise the relevance of machine learning in trading, dedicating Chapter 5 to its specific applications in the financial domain.
Blogs
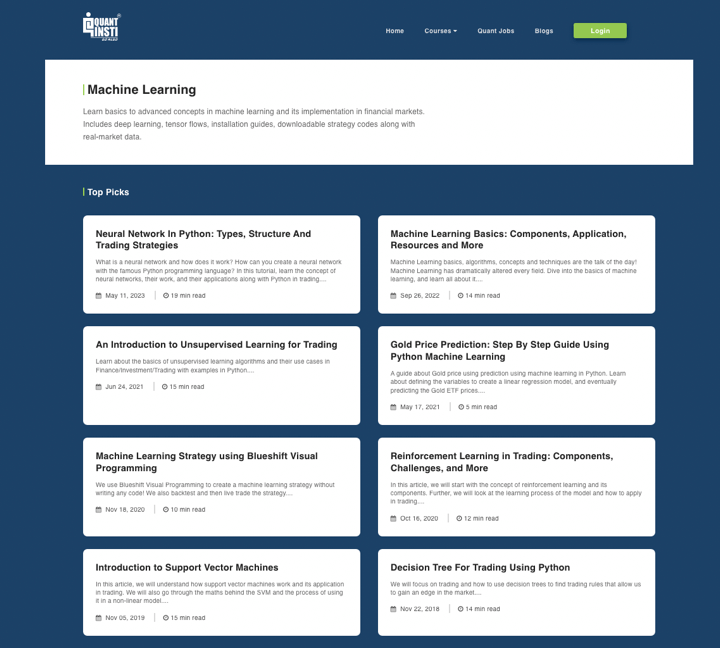
QuantInsti offers you a range of machine learning for trading blogs such as Trading Using Machine Learning in Python, Artificial Intelligence & Machine Learning in trading. You can find out which blog suits you depending on your level of expertise in machine learning.
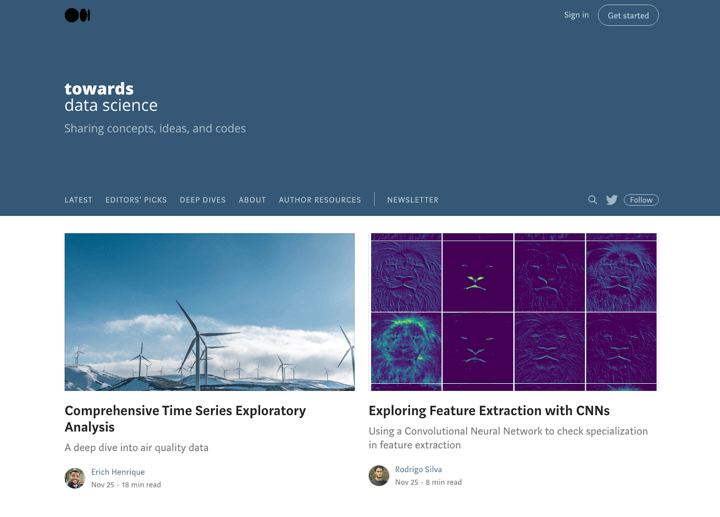
Here, you will find blogs such as Building RNN, LSTM, and GRU for time series using PyTorch, Deep reinforcement learning for automated stock trading and tips from an author whose machine learning trading algorithm outperformed the SP500 For 10 years.
3. Quantocracy
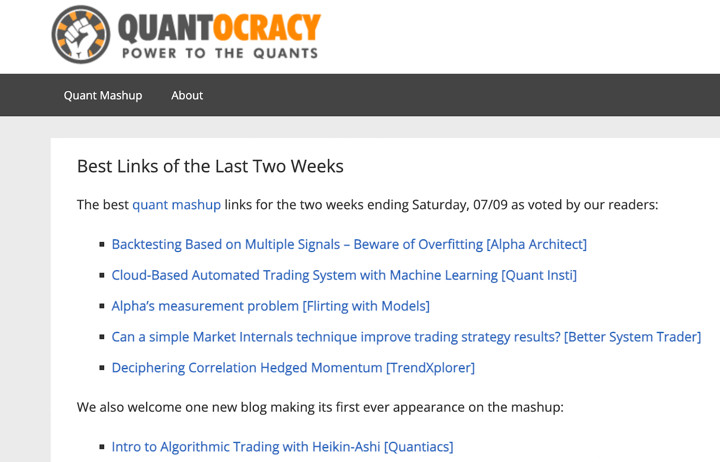
Quantocracy is a place where you can find a number of blogs on machine learning coming from different sources. They offer the user with a mashup of the most informative blogs on their website such as Metalabeling and the duality between cross-sectional and time-series factors [E.P. Chan] and Different methods for mitigating overfitting on Neural Networks.
4. Quantstart
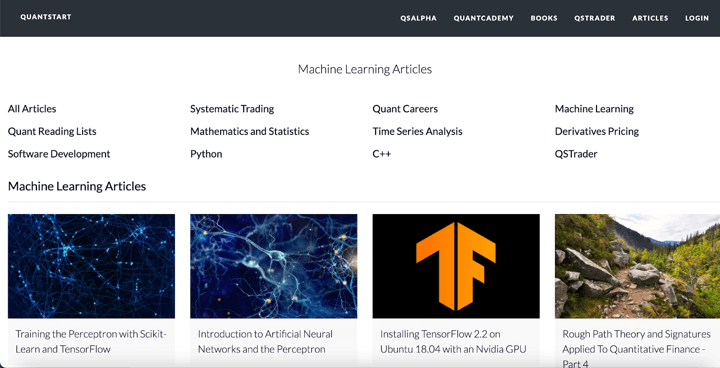
Quantstart has articles such as Beginner's Guide to Statistical Machine Learning and K-Means Clustering of Daily OHLC Bar Data that will help you with the application of machine learning in trading.
5. Medium
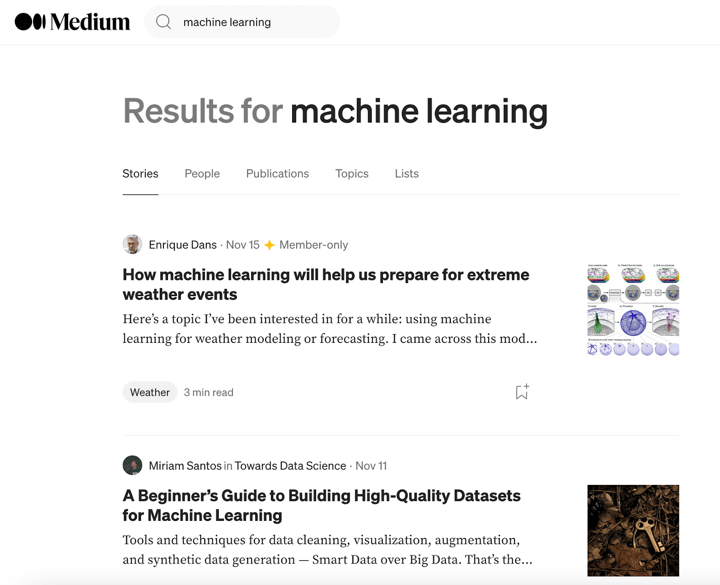
Blog articles such as Beginner’s guide to Machine learning, Big Data in Algorithmic Trading and various blogs on regression analysis offer you the information regarding such concepts like data, regression etc. used in trading. Find out more such blogs on the website.
Research papers:
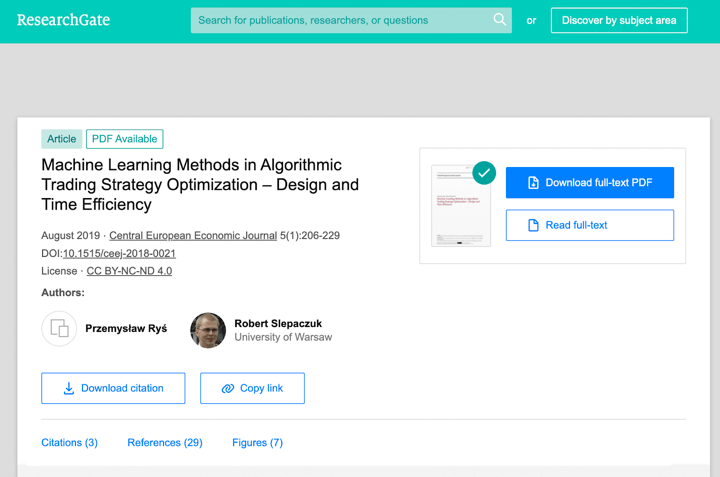
The aim of this research paper is to create and analyse the machine learning methods for trading. The methods here help solve the most important problems, such as the sensitivity of a strategy performance to little parameter changes. For instance, a sudden shift in the market trend is the change and strategy performance will be sensitive to it. But, with the machine learning methods here, the impact on the quality of strategy should not be huge. Hence, you get shortening of the computation time, without a substantial loss of strategy quality.
2. Machine learning for algorithmic trading
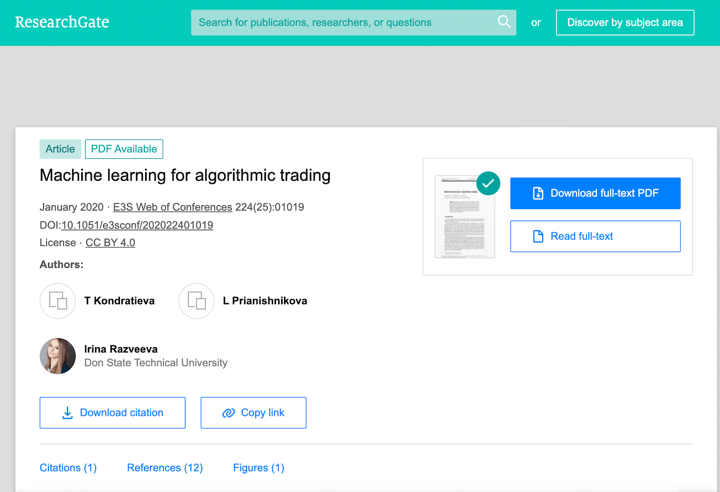
This paper by ResearchGate examines the theoretical and practical aspects of implementation of neural network in trading (a part of machine learning). The study suggests that high-precision forecasts and correct selection of parameters help to build favourable trading strategies.
3. Scalable Nearest Neighbor Algorithms for High Dimensional Data
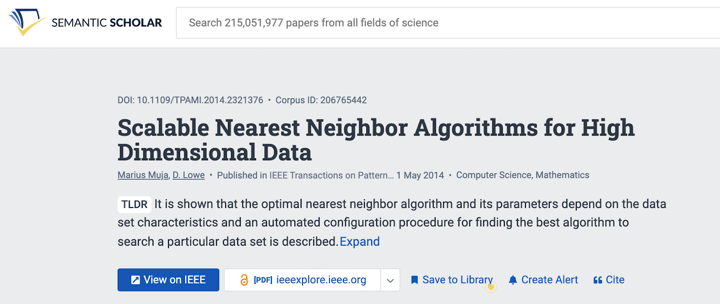
In this research paper, some advanced algorithms have been proposed. The distributed nearest neighbor framework here implies a significant help with the large data sets which would otherwise not fit in the memory of a machine. This is highly useful for backtesting in case of algorithmic trading using machine learning.
4. An Empirical Study of Machine Learning Algorithms for Stock Daily Trading Strategy
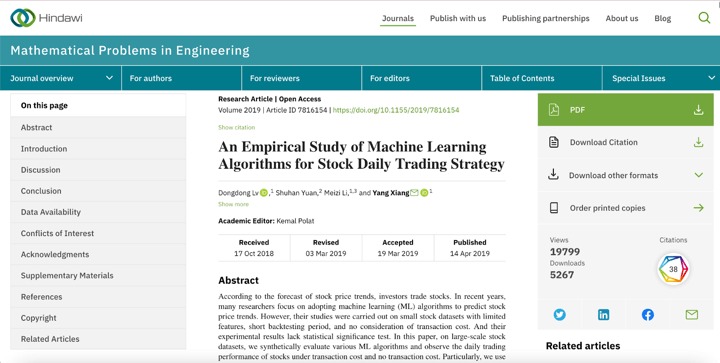
Recently, the researchers focus on adopting machine learning (ML) algorithms to predict stock price trends. In this paper, evaluation of various ML algorithms is done and daily trading performance of stocks under transaction cost and no transaction cost is observed. Moreover, large datasets are taken into account in this research paper to help with analysis from longer backtesting time periods (historical).
5. The virtue of simplicity: On machine learning models in algorithmic trading
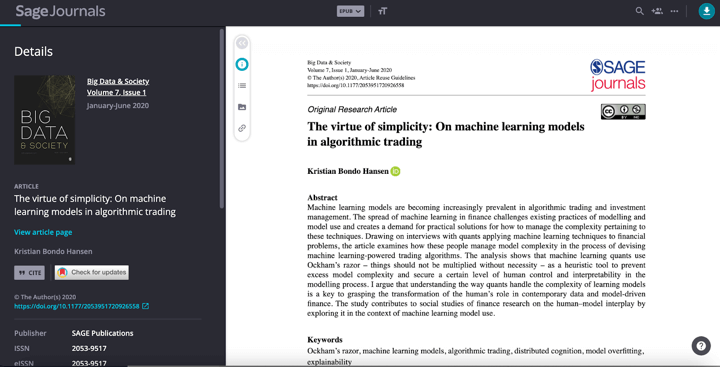
This paper examines how to manage model complexity in the process of devising trading algorithms using machine learning. The study contributes to social studies of finance research on the human–model interplay and uses it for creating machine learning models.
6. An Application of Deep Reinforcement Learning to Algorithmic Trading
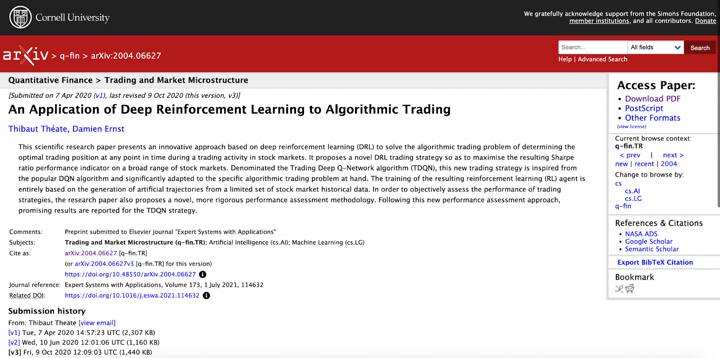
This scientific research paper presents an innovative approach based on deep reinforcement learning (DRL) to solve the algorithmic trading problem of determining the optimal trading position at any point in time during a trading activity in stock markets. It proposes a novel DRL trading strategy so as to maximise the resulting Sharpe ratio performance (an indicator) on a broad range of stock markets. Learn more in our free stock market beginner course.
Videos
This video talks about the introduction to machine learning for traders. It explains everything from basics of machine learning to implementation of machine learning in trading.
2. Leveraging Artificial Intelligence to Build Algorithmic Trading Strategies
This video brings to you a one hour webinar in which Mr. Tad Slaff, CEO of Inovance has explained how to include machine learning algorithms in a live trading strategy. Moreover, the book also talks about execution of live trading.
3. Machine Learning for Algorithmic Trading
There are 2 parts to the video here. The videos by Quant News serve as informative content for learning algorithmic trading with the help of machine learning. In these videos, you will see a step-by-step introductory process for implementing machine learning and how you can use machine learning algorithms for trading using Python.
- In part 1, you will learn the initial steps of using machine learning for algorithmic trading.
- In part 2, you will learn how to select the most important features to extract and clean your data.
In addition to all this, with these videos you will learn the process of tuning your parameters for better performance of your trading system.
To further enhance your understanding, consider enrolling in algo trading courses offered by Quantra, which complement the video content. These courses provide deeper insights into the integration of machine learning techniques in trading strategies, ensuring you not only grasp the theoretical concepts but also apply them effectively in practice.
4. Applying ML techniques to Trading Problems
Along with the do’s and don'ts in the application of machine learning for trading, this video describes a systematic approach to applying Machine Learning techniques to solve trading problems. This is not a tutorial on machine learning, but on how to adapt common ML techniques to develop profitable strategies.
5. Practical Tips For Algorithmic Trading (Using Machine Learning)
In this video, Jenia (an algorithmic trader) used machine learning tools to write his trading algorithm that now trades an initial $1M investment. He is talking about his approach and his main learnings.
6. Podcast with Dr. Ernest Chan: Predicting profitability using machine learning
This podcast offers you a comprehensive knowledge on predicting the profitability of trades using machine learning. This podcast video covers essential aspects of using machine learning during trading such as using conditional probabilities, risk management etc. with machine learning.
7. Machine learning for everybody
This is an approachable video on Youtube designed for accessibility. Here is an overview of the description on the course page.
Led by Kylie Ying, it embraces a hands-on, code-first methodology using Google Colab to build intriguing models. This course demystifies machine learning with topics like Introduction to ML, K-Nearest Neighbors, Naive Bayes, Logistic Regression, Linear Regression, K-Means Clustering, and Principal Component Analysis (PCA).
Conclusion
There are a lot of informative sources on machine learning for trading online. The best are researched and made accessible in this blog. Moreover, it is very much possible to find informative sources free of cost!
If you are interested in learning Machine Learning and its applications in trading, here's a highly-recommended track from Quantra - Learning Track: Machine Learning & Deep Learning in Trading - I. Perfect for beginners and experts, if you consider machine learning as an important part of the future in financial markets, you can't afford to miss this specialisation.
Author: Chainika Thakar (Originally written by Anupriya Gupta)
Note: The original post has been revamped on 21st December 2023 for accuracy, and recentness.
Disclaimer: All data and information provided in this article are for informational purposes only. QuantInsti® makes no representations as to accuracy, completeness, currentness, suitability, or validity of any information in this article and will not be liable for any errors, omissions, or delays in this information or any losses, injuries, or damages arising from its display or use. All information is provided on an as-is basis.