By Chainika Thakar and Shagufta Tahsildar
Decision trees are often used while implementing machine learning algorithms. The hierarchical structure of a decision tree leads us to the final outcome by traversing through the nodes of the tree. Each node consists of an attribute or feature which is further split into more nodes as we move down the tree. But how do we decide:
- Which attribute/feature should be placed at the root node?
- Which features will act as internal nodes or leaf nodes?
To decide this, and how to split the tree, we use splitting measures like Gini Index, Information Gain, etc. In this blog, we will learn all about the Gini Index, including the use of Gini Index to split a decision tree.
Find it all out with this blog which covers:
Offered by Dr. Ernest Chan, learn to predict markets and find trading opportunities using AI techniques
What is Gini Index?
Gini Index or Gini impurity measures the degree or probability of a particular variable being wrongly classified when it is randomly chosen.
But what is actually meant by ‘impurity’?
If all the elements belong to a single class, then it can be called pure. The degree of Gini Index varies between 0 and 1,
where,
'0' denotes that all elements belong to a certain class or there exists only one class (pure), and
'1' denotes that the elements are randomly distributed across various classes (impure).
A Gini Index of '0.5 'denotes equally distributed elements into some classes.
Terms similar to Gini Index for execution of decision tree technique
We are discussing the components similar to Gini Index so that the role of Gini Index is even clearer in execution of decision tree technique.
The very essence of decision trees resides in dividing the entire dataset into a tree-like vertical information structure so as to divide the different sections of the information with root nodes at the top.
In the decision tree model, each node is an attribute or the feature that contains necessary information (going sequentially downward) for the decision tree model. These are the necessary points to keep in mind while deciding each node of the decision tree model:
- Which features are to be located at the root node from where the decision tree will begin. This information at the root node should be the base of the entire information going forward. For instance, if we are going to create the decision tree model for a stock, we could mention the data (OHLCV) of the stock at the root node.
- Deciding which are the most accurate features to serve as the internal nodes (going vertically down the tree), also known as the leaf nodes.
Coming to the other terms that also lead to the execution of the decision tree technique, similar to the Gini Index, these are as follows:
- Splitting measures
- Information gain
Decision Trees in Financial Markets
Create a machine learning trading strategy using Decision Trees and ensemble methods
Splitting measures
With more than one attribute taking part in the decision-making process, it is necessary to decide the relevance and importance of each of the attributes. Thus, placing the most relevant feature at the root node and further traversing down by splitting the nodes.
As we move further down the tree, the level of impurity or uncertainty decreases, thus leading to a better classification or best split at every node. Splitting measures such as Information gain, Gini Index, etc. are used to decide the same.
Information gain
Information gain is used to determine which feature/attribute gives us the maximum information about a class.
- Information gain is based on the concept of entropy, which is the degree of uncertainty, impurity or disorder.
- Information gain aims to reduce the level of entropy starting from the root node to the leaf nodes.
Relevance of Entropy
Entropy is a measure of the disorder or the measure of the impurity in a dataset. The Gini Index is a tool that aims to decrease the level of entropy from the dataset.
In other words, entropy is the measurement of the impurity or, we can say, randomness in the values of the dataset.
A low disorder (no disorder) implies a low level of impurity. Entropy is calculated between 0 and 1. The number “1” signifies a higher level of disorder or more impurity.
Although there can be other numbers of groups or classes present in the dataset that can be greater than 1. In the case of machine learning (and decision trees), 1 signifies the same meaning, that is, the higher level of disorder and also makes the interpretation simple. Hence, the decision tree model will classify the greater level of disorder as 1.
Entropy is usually the lowest disorder (no disorder) means a low level of impurity and higher disorder (maximum disorder) means there is a high level of impurity. The entropy is measured to reduce the uncertainty that comes with more impurity.
In the image below, you can see an inverted “U” shape representing the variation of entropy in the graph. In the image, the x-axis represents the data values and the y-axis represents the value of entropy.
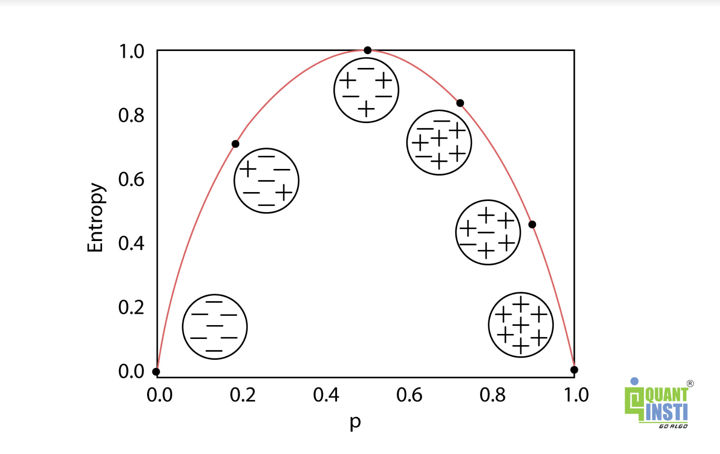
Offered by Dr. Ernest Chan, learn to predict markets and find trading opportunities using AI techniques
The graph above shows that the entropy is the lowest (no disorder) at two extremes (both left and right sides) and maximum (high disorder) in the middle of the graph or at the curve of the inverted “U” shape.
Therefore, at both extremes (left and right), there is no entropy (impurity) as each class has all the elements that belong to that class. On the other hand, in the middle, the entropy line stretches to the highest point to create a “U” shape where all the elements from two classes are randomly distributed which means there is entropy (impurity).
It is clear from our observation that both the extremes (left and right) are pure with no entropy.
Formula for Entropy
The formula for entropy, in order to find out the uncertainty or the high disorder, goes as follows:
$$E(S) = \sum_{i=1}^c - p_i log_2 p_i$$
where,
‘p’, denotes the probability of entropy and E(S) denotes the entropy.
Formula of Gini Index
The formula of the Gini Index is as follows:
$$Gini = 1-\sum_{i=1}^n (p_i)^2$$
where,
‘pi’ is the probability of an object being classified to a particular class.
While building the decision tree, we would prefer to choose the attribute/feature with the least Gini Index as the root node.
Example of Gini Index
Let us now see the example of the Gini Index for trading. We will make the decision tree model be given a particular set of data that is readable for the machine.
Now, let us calculate Gini Index for past trend, open interest, trading volume and return in the following manner with the example data:
Past Trend |
Open Interest |
Trading Volume |
Return |
Positive |
Low |
High |
Up |
Negative |
High |
Low |
Down |
Positive |
Low |
High |
Up |
Positive |
High |
High |
Up |
Negative |
Low |
High |
Down |
Positive |
Low |
Low |
Down |
Negative |
High |
High |
Down |
Negative |
Low |
High |
Down |
Positive |
Low |
Low |
Down |
Positive |
High |
High |
Up |
Calculation of Gini Index
We will now calculate the Gini Index with the following -
- Calculating the Gini Index for past trend
- Calculating the Gini Index for open interest
Calculating the Gini Index for past trend
Since the past trend is positive 6 number of times out of 10 and negative 4 number of times, the calculation will be as follows:
P(Past Trend=Positive): 6/10
P(Past Trend=Negative): 4/10
- If (Past Trend = Positive & Return = Up), probability = 4/6
- If (Past Trend = Positive & Return = Down), probability = 2/6
Gini Index = 1 - ((4/6)^2 + (2/6)^2) = 0.45
- If (Past Trend = Negative & Return = Up), probability = 0
- If (Past Trend = Negative & Return = Down), probability = 4/4
Gini Index = 1 - ((0)^2 + (4/4)^2) = 0
- Weighted sum of the Gini Indices can be calculated as follows:
Gini Index for Past Trend = (6/10)0.45 + (4/10)0 = 0.27
Decision Trees in Financial Markets
Create a machine learning trading strategy using Decision Trees and ensemble methods
Calculating the Gini Index for open interest
Coming to open interest, the open interest is high 4 times and low 6 times out of total 10 times and is calculated as follows:
P(Open Interest=High): 4/10
P(Open Interest=Low): 6/10
- If (Open Interest = High & Return = Up), probability = 2/4
- If (Open Interest = High & Return = Down), probability = 2/4
Gini Index = 1 - ((2/4)^2 + (2/4)^2) = 0.5
- If (Open Interest = Low & Return = Up), probability = 2/6
- If (Open Interest = Low & Return = Down), probability = 4/6
Gini Index = 1 - ((2/6)^2 + (4/6)^2) = 0.45
- Weighted sum of the Gini Indices can be calculated as follows:
Gini Index for Open Interest = (4/10)0.5 + (6/10)0.45 = 0.47
Calculating the Gini Index for trading volume
Trading volume is 7 times high and 3 times low and is calculated as follows:
P(Trading Volume=High): 7/10
P(Trading Volume=Low): 3/10
- If (Trading Volume = High & Return = Up), probability = 4/7
- If (Trading Volume = High & Return = Down), probability = 3/7
Gini Index = 1 - ((4/7)^2 + (3/7)^2) = 0.49
- If (Trading Volume = Low & Return = Up), probability = 0
- If (Trading Volume = Low & Return = Down), probability = 3/3
Gini Index = 1 - ((0)^2 + (1)^2) = 0
- Weighted sum of the Gini Indices can be calculated as follows:
Gini Index for Trading Volume = (7/10)0.49 + (3/10)0 = 0.34
Gini Index attributes or features
Attributes/Features |
Gini Index |
Past Trend |
0.27 |
Open Interest |
0.47 |
Trading Volume |
0.34 |
From the above table, we observe that ‘past trend’ has the lowest Gini Index and hence, it will be chosen as the root node for how the decision tree works.
Determining the sub nodes or the branches (features going down) of the decision tree
We will repeat the same procedure to determine the sub-nodes or branches of the decision tree.
We will calculate the Gini Index for the ‘positive’ branch of past trend as follows:
Past Trend |
Open Interest |
Trading Volume |
Return |
Positive |
Low |
High |
Up |
Positive |
Low |
High |
Up |
Positive |
High |
High |
Up |
Positive |
Low |
Low |
Down |
Positive |
Low |
Low |
Down |
Positive |
High |
High |
Up |
Calculating Gini Index of open interest for positive past trend
Open interest for positive past trend is high 2 times out of 6 and low 4 times out of 6 and the Gini Index of open interest for positive past trend is calculated as follows:
P(Open Interest=High): 2/6
P(Open Interest=Low): 4/6
- If (Open Interest = High & Return = Up), probability = 2/2
- If (Open Interest = High & Return = Down), probability = 0
Gini Index = 1 - (sq(2/2) + sq(0)) = 0
- If (Open Interest = Low & Return = Up), probability = 2/4
- If (Open Interest = Low & Return = Down), probability = 2/4
Gini Index = 1 - (sq(0) + sq(2/4)) = 0.50
- Weighted sum of the Gini Indices can be calculated as follows:
Gini Index for Open Interest = (2/6)0 + (4/6)0.50 = 0.33
Calculating Gini Index for trading volume
The trading volume is high 4 out of 6 times and low 2 out of 6 times and is calculated as follows:
P(Trading Volume=High): 4/6
P(Trading Volume=Low): 2/6
- If (Trading Volume = High & Return = Up), probability = 4/4
- If (Trading Volume = High & Return = Down), probability = 0
Gini Index = 1 - (sq(4/4) + sq(0)) = 0
- If (Trading Volume = Low & Return = Up), probability = 0
- If (Trading Volume = Low & Return = Down), probability = 2/2
Gini Index = 1 - (sq(0) + sq(2/2)) = 0
- Weighted sum of the Gini Indices can be calculated as follows:
Gini Index for Trading Volume = (4/6)0 + (2/6)0 = 0
Gini Index attributes or features
Attributes/Features |
Gini Index |
Open interest |
0.33 |
Trading volume |
0 |
We will split the node further using the ‘Trading Volume’ feature, as it has the minimum Gini Index.
Conclusion
Gini Index is a powerful measure of the randomness or the impurity or entropy in the values of a dataset. Gini Index aims to decrease the impurities from the root nodes (at the top of decision tree) to the leaf nodes (vertical branches down the decision tree) of a decision tree model.
You can learn all about decreasing the impurities going down the decision tree model with our course on Decision Trees offered by Dr. Ernest Chan. You will learn more about different splitting measures including Gini Index, Information Gain, etc. and also how to predict markets and find trading opportunities using AI techniques. Happy learning!
In case you are also interested in developing lifelong skills that will always assist you in improving your trading strategies. In this algo trading course, you will be trained in statistics & econometrics, programming, machine learning and quantitative trading methods, so you are proficient in every skill necessary to excel in quantitative & algorithmic trading. Know more about the EPAT course now!
Note: The original post has been revamped on 24th November 2022 for accuracy, and recentness.
Disclaimer: All investments and trading in the stock market involve risk. Any decision to place trades in the financial markets, including trading in stock or options or other financial instruments is a personal decision that should only be made after thorough research, including a personal risk and financial assessment and the engagement of professional assistance to the extent you believe necessary. The trading strategies or related information mentioned in this article is for informational purposes only.