Value at Risk (VaR) serves as a crucial tool in the financial landscape. This statistical measure quantifies potential losses in portfolios over a specified time horizon, offering a tangible understanding of risk with a defined level of confidence. And this comprehensive guide not only provides an introduction to value at risk but a lot more that will help you dive into it.
From optimising portfolios to regulatory compliance, VaR finds widespread application. However, the journey with VaR is not without challenges, including assumptions and oversimplified views.
Looking forward, promising trends like machine learning integration and dynamic risk modelling signal a future where VaR evolves to better understand and adapt to the ever-changing currents of financial risk.
This blog covers:
- What is Value at Risk?
- Key components of Value at Risk
- Value at Risk vs. Expected Shortfall
- Where is Value at Risk applied in trading?
- Trading styles where Value at Risk is applied
- Calculation of Value at Risk
- Value at Risk and its role in risk management
- Regulatory requirements of Value at Risk
- Challenges of using Value at Risk
- Tips to overcome the challenges of using Value at Risk
What is Value at Risk?
Value at Risk (VaR) is a statistical measure widely used in financial risk management to assess the potential loss on a portfolio of financial assets over a specific time horizon, with a certain level of confidence. In simpler terms, VaR quantifies the maximum amount of loss that a portfolio could suffer within a given time frame and probability.⁽¹⁾
Example of Value at Risk
VaR is expressed as a specific dollar amount and a confidence level, such as "There is a 5% probability that the portfolio will incur losses greater than $X over the next Y days." The calculation takes into account various factors, including the volatility of the financial instruments in the portfolio and the correlation between them.
Key components of Value at Risk
The calculation of Value at Risk (VaR) involves several key components that collectively provide a measure of the potential loss in a financial portfolio.
Here's a brief overview of each key component:
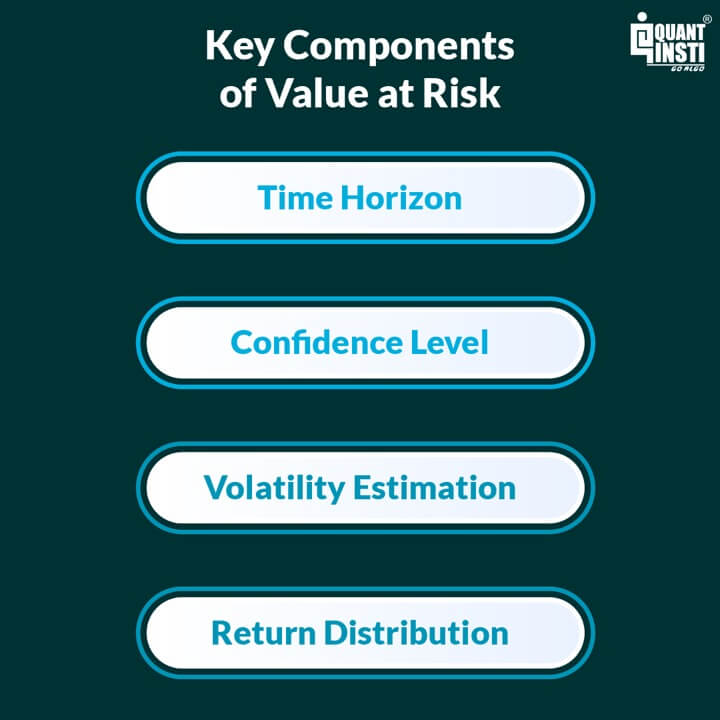
Time Horizon
The time horizon specifies the period over which VaR is calculated. It represents the length of time for which the risk is being assessed, such as one day, one week, or one month. The choice of time horizon is crucial, as it influences the sensitivity of the VaR measure to market movements.
Confidence Level
The confidence level, often expressed in terms of percentage (e.g., 95% or 99%), represents the level of certainty associated with the VaR estimate. In the case of historical simulation, (which will be discussed ahead in the blog) a 95% confidence level implies that there is a 5% chance of the actual loss exceeding the calculated VaR. The higher the confidence level, the higher the VaR value and vice versa.
Volatility Estimation
Volatility is a measure of the degree of variation of a trading price series over time. It is a crucial input in VaR calculations, reflecting the inherent uncertainty and risk in financial markets. Common methods for estimating volatility include historical volatility (based on past price movements), implied volatility (derived from option prices), and various statistical models.
Return Distribution
The return distribution implies the statistical distribution of potential returns (of the portfolio or asset). The choice of return distribution, often assumed to be normal but may include alternatives like the Student's t-distribution, affects the accuracy of VaR estimates. Non-normality in return distributions can be significant, especially during extreme market events.
These components collectively contribute to the quantification of VaR, providing a measure of the potential loss under specific conditions.
It's important to note that while VaR is a widely used risk measure, it has limitations, particularly in capturing tail risk in extreme events. Some risk management frameworks may also incorporate additional measures, such as Expected Shortfall (ES), to address these limitations and provide a more comprehensive view of risk.
Value at Risk vs. Expected Shortfall
Before seeing the differences between Value at Risk and expected shortfall, let us briefly see what the expected shortfall is.
Conditional Value-at-Risk, also known as Expected Shortfall (ES), is a risk assessment measure that quantifies the amount of tail risk in an investment portfolio. It is computed by taking a weighted average between the VaR value and the losses exceeding VaR.
As you can see below, CVaR is seen as an extension of VaR and is considered superior to VaR as it accounts for losses exceeding VaR. Therefore, you can use CVaR in your portfolio optimisation strategy to get a better idea of extreme losses and effectively manage your portfolio risk.
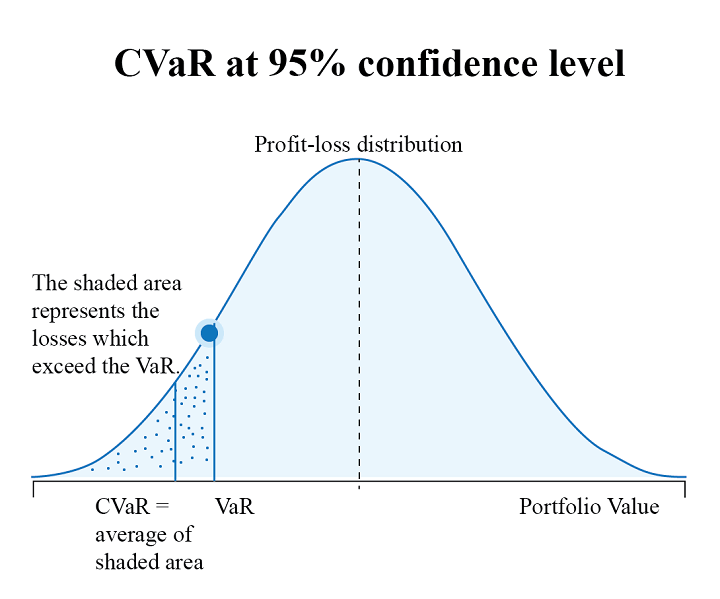
Now, let us find out the key differences between Value at Risk and expected shortfall (ES).⁽²⁾
Feature |
Value at Risk (VaR) |
Expected Shortfall (ES) |
Definition |
Quantifies the maximum potential loss at a given confidence level over a specific time horizon. |
Measures the average loss beyond the VaR threshold. |
Example |
A 95% VaR of $1 million implies there is a 5% chance of losing more than $1 million over a specified time horizon. |
An ES of $1 million at a 95% confidence level indicates that, in the worst-case scenario beyond the VaR threshold, the average loss would be $1 million. |
Focus |
Specific quantile at a confidence level, representing a threshold below which losses are not expected to exceed. |
Captures the average loss in the tail of the distribution, specifically beyond the VaR level. |
Interpretation |
Represents a dollar amount or percentage at risk with a certain probability. |
Represents the average amount of loss beyond the VaR level. |
Sensitivity to Extreme Events |
May underestimate the severity of extreme events as it focuses on a specific quantile. |
Specifically addresses the severity of extreme events by considering the entire tail. |
Shortcomings |
Can be less informative about the severity of extreme events. |
Requires more data and computational resources but offers a more comprehensive view. |
Regulatory Perspective |
Historically common in regulatory frameworks. |
Some regulators advocate its use as a complementary measure to VaR. |
Portfolio Management |
Commonly used for risk limits and capital allocation. |
Provides insights into risk for more conservative risk management strategies. |
Where is Value at Risk applied in trading?
Value at Risk (VaR) is a widely used risk management metric in the financial industry, and it is applied in various trading activities to assess the potential losses that a portfolio may face over a specific time horizon and with a certain level of confidence.
Below are some areas of application where Value at Risk is commonly applied in trading to assess potential losses:
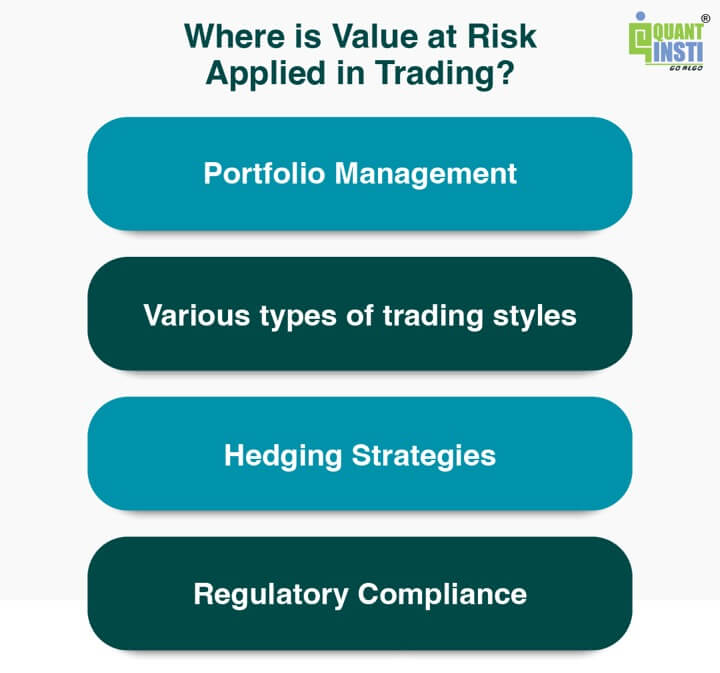
- Portfolio Management: Portfolio managers use Value at Risk to estimate the potential loss in the value of an investment portfolio over a specific time period. This helps them make informed decisions about asset allocation and risk exposure.
- Hedging Strategies: Value at Risk is used to evaluate the effectiveness of hedging strategies. It helps traders and investors assess how well their hedges protect against adverse market movements.
- Regulatory Compliance: Financial institutions are often required by regulators to calculate and report Value at Risk as part of regulatory capital requirements, especially under the Basel III framework.
Trading styles where Value at Risk is applied
Below are the various types of trading styles where Value at Risk is applied.
- Options Trading: Value at Risk is applied in options trading to evaluate the potential losses that may arise due to changes in the underlying asset's price, volatility, and other factors.
- Fixed Income Trading: Value at Risk is used to assess the impact of interest rate movements on the value of fixed income portfolios. This is crucial for bond traders and investors as it assesses the interest rate risk.
- Forex Trading: Value at Risk is applied to assess the potential losses in a portfolio due to fluctuations in exchange rates. Forex traders use Value at Risk to manage currency risk effectively.
- Commodity Trading: Traders in commodities markets use Value at Risk to estimate the potential losses resulting from changes in commodity prices.
Calculation of Value at Risk
Value at Risk (VaR) is a statistical measure used to quantify the potential loss on a financial asset or portfolio over a specified time period and with a certain level of confidence. There are different methods for calculating Value at Risk, and the choice of method depends on the characteristics of the portfolio and the assumptions made.
Three common methods for calculating Value at Risk are:
- Historical Simulation method to calculate Value at Risk,
- Variance-Covariance (Parametric) method to calculate Value at Risk, and
- Monte Carlo Simulation method to calculate Value at Risk.
You can check the detailed calculation part of the two most commonly used methods known as historical simulation and variance-covariance in the blog Value at Risk (VaR) calculation in excel and Python.
Briefly, below you can see how historical simulation and variance-covariance approaches are calculated.
Let’s start with the Variance-Covariance approach. The Variance-covariance is a parametric method which assumes that the returns are normally distributed.
In this method,
- We first calculate the mean and standard deviation of the returns.
- According to the assumption, for a 95% confidence level, the Value at Risk is calculated as a mean -1.65 * standard deviation.
- Also, as per the assumption, for a 99% confidence level, the Value at Risk is calculated as mean -2.33* standard deviation.
Please note that the above mentioned figures are based on a subjective assumption ⁽²⁾.
Moving on, the steps for Value at Risk calculation using the Historical simulation approach are as follows:
- Similar to the variance-covariance approach, first we calculate the returns of the stock Returns = Today’s Price - Yesterday’s Price / Yesterday’s Price
- Sort the returns from worst to best.
- Next, we calculate the total count of the returns.
- The VaR(90) is the sorted return corresponding to 10% of the total count.
- Similarly, the VaR(95) and VaR(99) are the sorted returns corresponding to the 5% and 1% of the total count respectively.
Value at Risk and its role in risk management
Let's delve into the Value at Risk (VaR) and its role in risk management.
Imagine you're steering a ship through a vast and unpredictable sea. Your goal is to navigate through waves and storms, ensuring that your vessel reaches its destination safely. In the financial world, that sea is the market, and Value at Risk is your compass, guiding you through the uncertainties.
The Financial Compass
Value at Risk is like a financial compass that helps institutions and investors gauge the potential loss they might face in their investment portfolios. It's not a crystal ball predicting the future, but rather a tool that estimates the level of risk given current market conditions.
Quantifying Risk
Let's say you have a portfolio of stocks and bonds. Value at Risk allows you to put a number on the potential loss your portfolio might experience over a specified time period, let's say one day or one week. This numerical value represents the downside risk within a certain confidence level, typically 95% or 99%. So, it's like saying, "There's a 95% chance that our losses won't exceed this amount."
Managing Diversification
Value at Risk is particularly handy when you're managing a diverse portfolio. It helps you understand how different assets contribute to the overall risk. For instance, if you have a mix of stocks and bonds, Value at Risk lets you see which asset class has a higher potential for volatility and how they interact. This insight is crucial for optimising your portfolio, ensuring you're not overly exposed to a particular type of risk.
Summary
In essence, Value at Risk is a valuable tool in the risk management toolkit. It doesn't eliminate risk, but it gives you a sense of the waters you're navigating and helps you make strategic decisions to sail through the financial seas with confidence.
Regulatory requirements of Value at Risk
Regulatory requirements often play a significant role in shaping risk management practices within the financial industry. Value at Risk (VaR) has historically been a key metric in regulatory frameworks, providing a quantitative measure of the potential downside risk of financial portfolios.
Here's a brief overview of the relationship between regulatory requirements and VaR:
- Basel Accords: The Basel Committee on Banking Supervision, through its Basel Accords ⁽³⁾(Basel I, Basel II, and Basel III), has outlined regulatory frameworks for banking institutions. Value at Risk has been used as a risk measure for regulatory capital adequacy calculations.
- Stress Testing and Backtesting: Regulators often require financial institutions to complement Value at Risk with stress testing and backtesting. Stress tests assess the impact of extreme events, and backtesting evaluates the accuracy of Value at Risk models against historical data.
- Pillar 2 Requirements: Under Basel II and III, Pillar 2 addresses qualitative aspects of risk management. Regulatory authorities may assess the overall risk management framework of banks, including the appropriateness of Value at Risk models and their integration into broader risk management practices.
- Supervisory Review and Evaluation Process (SREP): Regulatory authorities, as part of the SREP process, evaluate banks' risk management practices. Value at Risk models and their effectiveness in capturing and managing market risk are key components of this evaluation.
- Enhancements and Evolving Standards: Regulatory requirements for risk management, including the use of Value at Risk, continue to evolve. Institutions are expected to stay current with these changes and enhance their risk management frameworks accordingly.
Challenges of using Value at Risk
Now, let us find out the challenges which a trader might face while using Value at Risk and these are:
- Assumption of Normal Distribution: Value at Risk often assumes a normal distribution of asset returns, which may not hold during extreme market conditions or financial crises.
- Correlation and Diversification Assumptions: Value at Risk models may oversimplify the correlation structure between different assets, leading to underestimation of risk, especially in times of correlated market movements.
- Static Nature of Value at Risk: With Value at Risk, you typically get a snapshot of risk at a specific point in time, ignoring dynamic changes in market conditions and evolving risks.
- Model Sensitivity: Value at Risk outcomes are sensitive to the choice of model parameters and historical data periods, making it challenging to create a universally accurate and reliable model.
- Tail Risk and Black Swan Events: The result may underestimate the risk of extreme events (tail risk) or fail to capture rare, unpredictable events (black swan events), leading to inadequate risk coverage.
- Lack of Consideration for Market Illiquidity: VAR models may not adequately account for the illiquidity of certain assets, especially during stressed market conditions, potentially underestimating the true risk.
- Risk Horizon and Time Frame: The results of Value at Risk are highly dependent on the chosen time horizon, and different time frames may yield significantly different risk estimates.
- Model Complexity and Data Requirements: Implementing advanced Value at Risk models requires substantial computational resources and historical data, which may not be readily available or may be subject to data quality issues.
- Behavioural Factors and Market Shocks: Value at Risk models often struggle to incorporate behavioural factors and may not adequately capture market shocks driven by investor sentiment or sudden shifts in market perceptions.
Tips to overcome the challenges of using Value at Risk
Here are some useful tips for overcoming the challenges mentioned above.
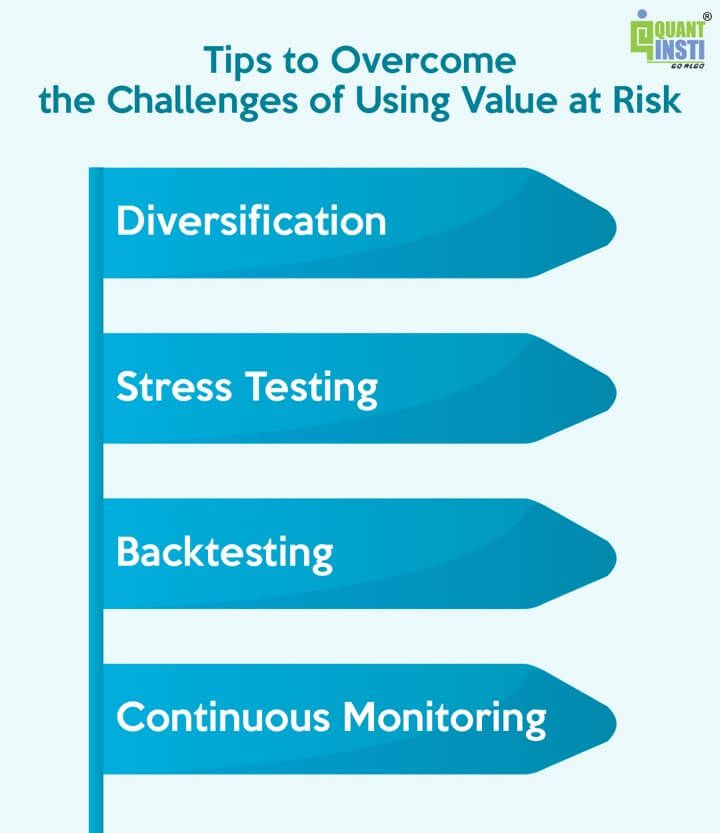
- Diversification: Mitigate risk by diversifying portfolios across different asset classes, reducing dependence on a single market. Diversified portfolios tend to exhibit more stable and consistent returns over time. This stability aids in creating more reliable risk estimates, which is essential for the accuracy of Value at Risk calculations.
- Stress Testing: Complement Value at Risk with stress tests with the help of computational methods such as Monte carlo simulation. This stress testing will allow you to assess how portfolios perform under extreme conditions, providing insights into extreme events or tail risk such as the 2008 global financial crisis and COVID-19 pandemic.
- Backtesting: Regularly backtest Value at Risk models using historical data to validate their accuracy and adjust parameters as needed.
- Continuous Monitoring: Regularly reassess and update risk models, considering shifts in market dynamics and incorporating new information to maintain relevance.
By incorporating these tips, traders can strengthen their risk management frameworks and navigate the challenges associated with using Value at Risk more effectively.
Conclusion
In summary, Value at Risk (VaR) is like a trustworthy guide helping traders navigate the uncertain seas of the market. It puts a number on possible losses in portfolios, giving a clear picture of risk with a certain confidence level. From managing portfolios to dealing with regulations, Value at Risk has many uses, making it a key player in understanding financial risk.
You can explore more about value at risk and other risk management methods with our course on Quantitative Portfolio Management. Recommended for portfolio managers and quants, this course is designed for those who wish to construct their portfolio quantitatively, generate returns and manage risks effectively. In this course, you will also learn different portfolio management techniques such as Factor Investing, Risk Parity and Kelly Portfolio, and Modern Portfolio Theory.
Author: Chainika Thakar
Disclaimer: All data and information provided in this article are for informational purposes only. QuantInsti® makes no representations as to accuracy, completeness, currentness, suitability, or validity of any information in this article and will not be liable for any errors, omissions, or delays in this information or any losses, injuries, or damages arising from its display or use. All information is provided on an as-is basis.