How can one keep up with the changing trends in risk management in trading? How can someone manage risks better?
Risk management in trading might be an underrated concept, but it is an extremely integral part of algorithmic trading. Risk management in trading is not only about portfolio optimisation, hedging, strategy planning etc while creating your portfolio.
With the contemporary practice of algorithmic trading, risk management now also implies ensuring no risks concerning other aspects such as data quality, technology, and much more!
This blog covers:
Risk management around trading activity
Risk management, in trading, takes place for creating the trading portfolio with a strategy and also around the trading activity. In this blog, we will discuss the risk management around trading activity.
Below you can see an illustration of the architecture for algorithmic trading:
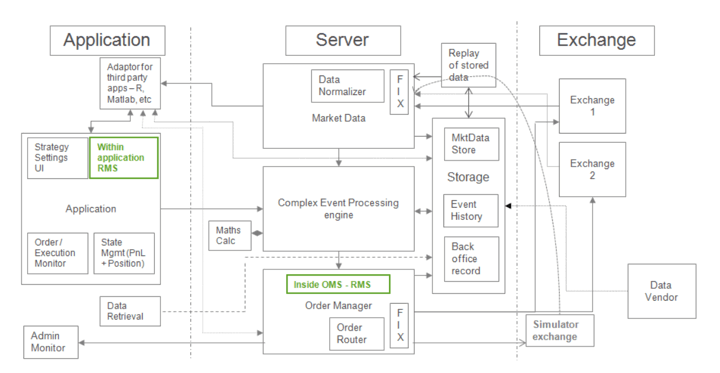
You can see in the above image that the order is placed through the application in a computer language such as R, MATLAB, Python etc.
Then, the order is executed via the application and it goes through the server where all the necessary events take place such as data retrieval, calculation according to the strategy inputted, etc. Then the data is sent to the exchange as per the strategy.
In the entire process of algorithmic trading, there are several risks related to the network of the system, technology, proper working of human resources etc. that we will speak about further.
Let us now see these necessary points for risk management to ensure smooth trading practice in detail.
Where is risk management required?
As we saw in the image above, the order signals generated are executed via an API which, then, works to send the information regarding order execution to the exchanges.
This is done by retrieving the information of trade orders via your programming language (R, Python etc.). As an algorithmic trader, you must ensure proper risk management practices are followed for the API to work accurately.
Traditionally trading operations used to focus on the following risks:
Market risk or systematic risk
Market risk comes about due to the failure of the entire market instead of an industry or company due to some factors. These factors can be broadly categorised into social, political and economic issues.
For instance, interest risk, inflation risk or any such market risk to the firm. This kind of risk befalls the entire industry. The solution to this risk is measuring the volatility through VIX indicator and exiting the high risk assets when volatility is high.
Credit or Counterparty risk
Credit risk is related to the creditworthiness of an individual, a corporation or a sovereign government. To maintain its creditworthiness, a company needs a good credit rating for which the assessment is done by agencies such as Standard & Poor’s, Moody’s, or Fitch. The credit rating helps when you as a trader or an organisation need to raise funds for your trades.
Liquidity risk
Risk to do with liquidity refers to the ability and ease with which assets can be converted into cash without affecting the current asset price in the market to a great extent.
Market liquidity refers to the extent to which a market allows assets such as stocks, bonds, or derivative products, to be bought and sold without paying a huge bid-ask spread. Having such liquid assets helps a trader with managing the liquidity risk.
Regulatory risk
The regulatory risk refers to the risk of the laws and regulations getting changed which can adversely affect a trader’s trading strategy. The regulatory laws and regulations have been revised after the advent of algorithmic trading practices which we will discuss further.
However, with the advent of automated trading this focus has shifted to the following along with the above:
- Operational risk
- Scalability risk
- Technological risk
- Human resource risk
- Regulatory risk related to automated trading
Operational risk
Operational risk (OR) is the risk that a trading house faces due to a failed internal process or system/network flaw.
Operational risk involves a wide range of “non-financial problems” such as:
- Technology risk where a computer system or network architecture is not updated, or the personnel is incompetent.
- Lack of structured risk policies.
- Process-related risks such as the possibility of error in information processing, data transmission, data retrieval and inaccuracy of result or output.
- Other risks include lack of proper monitoring of risk, employee’s or management’s involuntary errors, employee or management fraud or criminal activities.
- Finally, it could include losses due to natural disasters, terrorism and so on.
Scalability risk
The traders face maximum risks due to scalability. The scalability risk states that a particular enterprise, be it s hedge fund, a mutual fund, or any other institutional trading firm is at risk of not scaling up in challenging situations.
For instance, a hedge fund might not be able to increase its capital allocation in the market because of a fewer number of people holding its shares in the market. Until and unless the number of shareholders is increased, the opportunities to buy and sell the shares and to create profits are the bare minimum.
Technological risk
This is an event caused by malfunctioning of the technological structure and/or human error in handling or operating a technology/machine. For instance, one of the reasons for the Flash crash (2010) is believed to be the technological glitch in the reporting of prices on US exchanges.
Human resource risk
This is one of the integral risks in the era of algorithmic trading and poses risk to the trading firms such as hedge funds, proprietary trading firms etc.
For successful algorithmic trading, the employment of human resources with a sound educational background in finance, computer science etc. plays an important role.
Apart from the background check concerning education, there has to be a reliable employee management system for:
- Regular monitoring of productivity relating to the checks on certain parameters that the employees deploy such as stop orders, a particular trading volume, a strategy such as momentum, scalping etc.
- Ensuring that employees are compliant with laws and regulations about the regulatory structure of algorithmic trading.
Regulatory risk related to automated trading
Regulatory risk implies the risk of being punished by the government of the particular country you indulge in the trading practices if you do not follow the laws and regulations.
Usually, the government authority requires you to get all your strategies approved by the exchange. A half-yearly and an annual audit are both also required by the regulator, exchange and independent auditors.
Once you have an automated trading strategy you need to execute it in a Mock Trading environment.
While submitting a strategy to the exchange for approval following are the risk management checks that one needs to show:
- Manual orders are disabled for auto-trading systems
- The order should be within x% of the last price
- For each instrument, an order size freeze limit is set
- The order should not breach the circuit limit (daily price range) of an instrument
- FIIs cannot trade in a select set of stocks (RBI directed)
- Cannot trade derivatives to increase Open Interest beyond a threshold
- An overnight long position that is available per share for selling
- Automated trading to be enabled for a select list of instruments only
- Cannot send buy orders if Index moves up beyond a point. Likewise for sell orders
- The maximum position that a client can have in a particular stock
- If a threshold of the available margin is reached, then the application should not send orders to increase the position further
- Net Position value per instrument
- Max Order Value
Simply knowing the building blocks is not enough; one should know what strategies others or competitors are using.
To give you this insight we have a section called “Financial Computing & Technology” in our Executive Programme in Algorithmic Trading. This will certainly inspire you to design your trading strategies; different concepts can be used to devise your strategies.
How to ensure a good risk management practice?
To ensure good risk management practice, the following help:
- Knowledge - With the right set of knowledge, an algorithmic trader can build all the requirements discussed above. If you know what is needed for your strategy to work uninterruptedly, you can arrange for the same.
- Set up - A set-up with everything - technology, hardware, software etc. in place can make a whole lot of difference. Working with a correct setup will ensure that you keep a check on the risk management practices.
- Reliable team for those working in a team setup - With a team to rely on an algorithmic trader can segregate the responsibilities to different teams. This way, the trader can make sure that each team performs to ensure that risk management practices are being followed.
- Using Artificial Intelligence (AI) - A good risk management practice can be ensured using AI, specifically Machine Learning (ML). By inputting the machine learning model with tasks such as-
- Reviewing potential employees’ background for good human resource management, ensuring etc. the model can be brought into use for managing risks in the area of human resource management.
2. Regularly monitoring the productivity of the employees
3. Ensuring that the employees follow the rules and regulations stated by the government for algorithmic trading practices.
But, the most important is the capital to keep all the necessities in place for a good risk management practice to be followed.
Conclusion
To ensure a good risk management practice, one needs to make some effort to find out what is needed for keeping the risks at bay. Once figured out, the risk management gets easier to implement and your successful trading strategy can keep bringing you the returns you foresee without an interruption.
For such a successful trading experience, a trader must keep following good risk management practices for a happy trading journey!
You too can enjoy trading with your algorithmic trading strategies, with the right guidance as provided in this course on position sizing in trading. You can learn all about money management, find out the inherent risks in the financial markets and incorporate all learnings in the course to create a conservative position sizing framework. Be sure to check it out!
Note: The original post has been revamped on 1st August 2022 for accuracy, and recentness.
Disclaimer: All investments and trading in the stock market involve risk. Any decision to place trades in the financial markets, including trading in stock or options or other financial instruments is a personal decision that should only be made after thorough research, including a personal risk and financial assessment and the engagement of professional assistance to the extent you believe necessary. The trading strategies or related information mentioned in this article is for informational purposes only.