By Vivek Jain
Statistical arbitrage pair trading involves identifying pairs of stocks that exhibit mean-reverting behavior. This strategy is widely used to exploit temporary deviations in the relative prices of the pairs. This project explores the application of statistical arbitrage in different sectors of the Indian market, motivated by the potential for market-neutral profits and risk diversification.
This “Statistical Arbitrage Pairs Trading” strategy in NSE-listed stocks of different sectors leverages quantitative precision and risk hedging to make data-driven trading decisions. By identifying cointegrated stocks from various sectors, the strategy focuses on the statistical relationship between asset pairs, specifically their spread or hedge ratio, to minimize market-wide risk.
The hedge ratio is determined using Ordinary Least Squares (OLS) regression, which helps balance positions between the two assets. Spreads are calculated and tested for stationarity using the Augmented Dickey-Fuller (ADF) test, selecting pairs with at least 90% statistical significance.
The strategy is executed by going long when the spread falls below a predefined threshold and closing the position when it reverts to the mean. Conversely, short positions are opened when the spread exceeds the threshold and closed once the spread returns to the mean. This method enhances discipline, reduces emotional bias, and provides a more robust and reliable approach to market-neutral trading.
This article is the final project submitted by the author as a part of his coursework in the Executive Programme in Algorithmic Trading (EPAT) at QuantInsti. Do check our Projects page and have a look at what our students are building.
Other EPAT Project publications on Statistical Arbitrage trading strategy are listed below.
- Statistical Arbitrage Strategy In R - By Jacques Joubert [EPAT PROJECT] (Year: 2016)
- Pair Trading – Statistical Arbitrage On Cash Stocks [EPAT PROJECT] (Year: 2017)
- Statistical Arbitrage: Pair Trading In The Mexican Stock Market [EPAT PROJECT] (Year: 2017)
- Statistical Arbitrage: Pair Trading In The Brazilian Stock Market [EPAT PROJECT] (Year: 2017)
- Dynamic Selection of Pairs for Statistical Arbitrage (Year: 2022)
About the Author
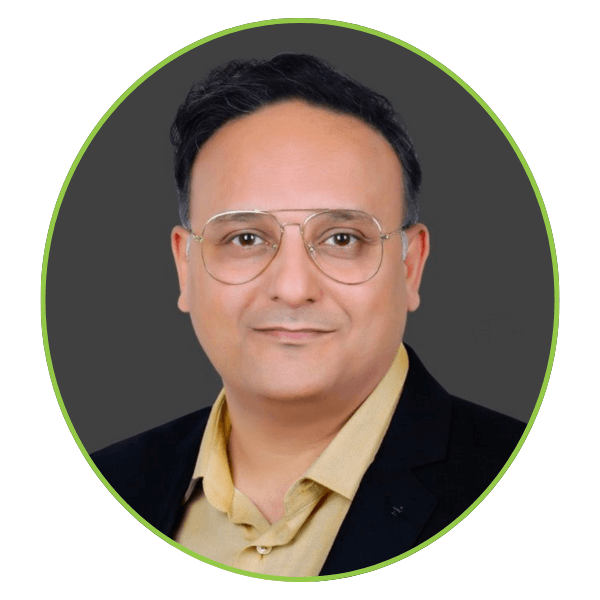
Vivek Jain is an EPAT alumni and a Certified Financial Technician (CFTe) accredited by IFTA, USA. He has also completed all levels of the Chartered Market Technician (CMT, USA) program. With over a decade of investment experience and nearly five years of full-time trading, he applies advanced technical analysis and quantitative methods to deliver superior performance.
He participated in the CMT Association’s Global Investment Challenge in August 2023 and September 2022, where he successfully qualified out of more than 1,000 registrants from 47 countries and 45 universities by trading S&P 500 stocks.
Achieved a top 3% ranking with a +3.21% net gain, equating to an absolute gain of $8,024.20, in one month by trading cryptocurrency, SPX, FX, and commodity futures during TradingView's global competition, "THE LEAP Nov '24," competing against over 59,000 participants
Specializing in designing and implementing systematic portfolio trading systems, he is currently focused on developing advanced mean reversion strategies and quantitative long/short strategies, utilizing sophisticated statistical techniques to enhance returns and optimize risk management.
In a recent project for a multinational corporation, Vivek built a Mutual Fund ranking system in Python, integrating historical NAVs and multiple performance metrics. His deep market knowledge and technical expertise enable him to excel in complex, data-driven environments.
Currently serving as a "Technical & Quantitative Analyst" at Kedia Capital, specializing in the development and implementation of quantitative strategies to deliver superior risk adjusted returns.
EPAT batch: #60
Certification status: Certification of Excellence
Mentor: Rekhit Pachanekar
Connect with me: https://www.linkedin.com/in/vivek-jain-cfte-70894324a/
Data Mining
Historical price data for stocks in different sectors of the Indian market is sourced from Yahoo Finance. The data includes adjusted closing prices for selected pairs of stocks spanning from January 1, 2008, to December 31, 2014. The data is downloaded and processed using the yfinance Python library.
Data Analysis
The project involves the following steps:
1. Pair Selection: Identifying pairs of stocks within the same sector that are likely to be cointegrated.
2. Cointegration Testing: Applying the Augmented Dickey-Fuller (ADF) test on the spread to verify the cointegration of pairs.
3. Spread Calculation: Calculating the spread between the cointegrated pairs.
4. Trading Signals: Generating trading signals based on the spread's mean-reverting behavior.
Key Findings
• Certain pairs within sectors demonstrate significant cointegration, validating the potential for pair trading. The spread between cointegrated pairs tends to revert to the mean, creating profitable trading opportunities.
• In some stocks, even when the p-value is significant, the overall strategy is not profitable.
During our testing period, the Bollinger Band strategy was found to be more effective than the Z-score strategy.
Challenges/Limitations
• The accuracy of cointegration tests and trading signals is influenced by market volatility and external factors.
• Execution risk and transaction costs may affect the real-world profitability of the strategy.
• Fundamental differences among stocks within certain sectors, such as Pharma, may hinder the identification of profitable pairs.
Implementation Methodology
The project is implemented using Python, leveraging libraries such as pandas for data manipulation, numpy for numerical operations, statsmodels for statistical testing, and yfinance for data retrieval. The methodology involves:
1. Downloading Data: Retrieving historical price data for selected stocks.
2. Calculating Cointegration: Using the ADF test to identify cointegrated pairs.
3. Calculating Spreads: Computing the spread between cointegrated pairs.
4. Generating Signals: Implementing the Bollinger Band and Z-score strategies to generate buy and sell signals.
5. Calculating Returns: Computing log returns for the strategy and evaluating performance.
Conclusion
The statistical arbitrage pair trading strategy offers a systematic approach to trading pairs of stocks within the Indian market. While it shows potential, the strategy's effectiveness varies across sectors and individual pairs. Further refinement and testing are required to enhance its robustness and applicability in real-world trading scenarios.
File in the download
The Python codes for implementing the strategy are provided, including data download, cointegration testing, spread calculation, signal generation, and performance analysis.
Next steps
If you are interested in learning more about Mean Reversion & Statistical Arbitrage, read the blogs here:
- Arbitrage Strategies: Understanding Working of Statistical Arbitrage
- Mean Reversion Strategies: Introduction, Trading, Strategies and More
- Mean Reversion in Time Series: What it is and Trading Strategies
- Pairs Trading for Beginners: Correlation, Cointegration, Examples, and Strategy Steps
- Hurst Exponent: Calculation, Values and More
Want to know how EPAT equips you with skills to build your trading strategy in Python? Check out the EPAT course curriculum to find out more.
Understand about different strategy paradigms so that you can create the right strategy for a specific market regime.
- Momentum Trading Strategy
- Mean Reversion Strategies
- Volatility Trading Strategy
- Factor Investing
- Day Trading Strategy
Disclaimer:The information in this project is true and complete to the best of our Student’s knowledge. All recommendations are made without guarantee on the part of the student or QuantInsti®. The student and QuantInsti® disclaim any liability in connection with the use of this information. All content provided in this project is for informational purposes only and we do not guarantee that by using the guidance you will derive a certain profit.