Stepping into the dynamic realm of financial markets necessitates a comprehensive understanding of the strategic manoeuvres deployed by traders. 'Types of Trading Strategies' unfolds as an exploration into the diverse methodologies that guide investors in making sound decisions, managing risks, and optimising outcomes.
The different trading strategies, pivotal in the trading landscape, are crucial for a successful trading experience. From fundamental analysis to technical indicators, this journey unveils the multifaceted approaches that professionals employ.
This comprehensive overview seeks to demystify the intricate world of trading strategies, offering a nuanced perspective on how these tools contribute to navigating the complexities of financial markets with precision and astuteness.
This informative blog covers:
- What is a trading strategy?
- Asset classes in trading
- Components of a trading strategy
- Types of trading strategies
- Machine Learning based trading strategies
- Methods of creating trading strategies
- Portfolio of trading strategies
- Innovations in trading strategies
- Common mistakes to avoid in different trading strategies
- How to choose the right trading strategy?
What is a trading strategy?
A trading strategy is a systematic plan to guide decisions in buying or selling financial instruments. Its purpose is to maximise trading returns by identifying market opportunities while managing risks.
Trading strategies involve specific rules, criteria, or indicators for determining entry and exit points. These plans can be based on technical, fundamental, or quantitative analysis.
Asset classes in trading
Asset classes in trading refer to broad categories of financial instruments that share similar characteristics and are subject to similar market dynamics. These classes provide investors and traders with diverse options for building portfolios and managing risk.
Here are some common asset classes in trading:
- Equities (Stocks): Ownership shares in publicly traded companies, representing a claim on a portion of the company's assets and earnings.
- Bonds (Fixed-Income Securities): Debt instruments where investors lend money to governments, municipalities, or corporations in exchange for periodic interest payments and the return of principal at maturity.
- Forex (Foreign Exchange): The global marketplace for trading currencies, involving the exchange of one currency for another with the goal of making a profit from currency value fluctuations.
- Commodities: Physical goods such as gold, silver, oil, agricultural products, and more, traded on commodity exchanges.
- Real Estate: Investments in physical properties, including residential, commercial, and industrial real estate.
- Derivatives: Financial instruments derived from an underlying asset, such as options and futures contracts, allowing traders to speculate on price movements without owning the actual asset.
- Cryptocurrencies: Digital or virtual currencies that use cryptography for security, with Bitcoin and Ethereum being notable examples.
- Cash and Cash Equivalents: Highly liquid and low-risk assets, including treasury bills, certificates of deposit, and money market instruments.
Suggested read: Algorithmic Trade Execution in different Asset Classes
Components of a trading strategy
The following are components of a trading strategy:
- Trade universe
- Entry and exit logic
- Risk management
Trade Universe
The realm of types of trading strategies extends to the trade universe, encompassing diverse products and markets. A broad array of tradable products, including futures, options, and equities, facilitates trading across various markets such as currency, commodities, stocks, and cryptocurrencies. Each trading product and market introduces unique risks and trade dynamics, emphasising the importance of selecting a strategy tailored to the specific characteristics of the chosen assets.
Entry and Exit Logic
Central to effective types of trading strategies is the establishment of entry and exit logic. These are predefined conditions dictating when to buy or sell a stock. The analysis method employed within the trading strategy delineates specific entry and exit price levels, guiding traders in executing precise and informed transactions.
Risk Management
A critical facet of types of trading strategies involves robust risk management. Capital allocation and implementing stop-loss mechanisms constitute pivotal elements. Capital allocation determines the amount of capital assigned to each trade, while stop-loss serves to curtail the risk associated with a particular transaction. Following the design of a trading strategy, comprehensive backtesting is undertaken to evaluate and comprehend its performance.
Before progressing further, you must explore the landscape of the 15 most popular algo trading strategies. These strategies, widely adopted by traders and investors, are instrumental in automating trading decisions, showcasing the evolving landscape of algorithmic approaches within the realm of types of trading strategies.
Types of trading strategies
Trading strategies can be broadly classified into four types which are⁽¹⁾:
- Trend trading strategies
- Mean-Reverting strategies
- Carry trade strategies
- Event-based trading strategies
Trend trading strategies
The trend following strategy generates entry and exit conditions according to the trend of the stock. According to the trending trading strategy, an asset is bought during its uptrend and is shorted during the downtrend, assuming the price continues in the direction of the trend. And, the trade is exited once the trend reverses.
Using technical analysis, a trend trading strategy is designed based on indicators like moving average crossovers, RSI indicator, and average directional index (ADX). The following is an example of a trend trading strategy created using technical analysis.
Moving Average Crossover
When the stock is trending up, the moving averages of the price angle up and trends higher along with the price. According to the moving average crossover strategy, a stock will be bought when the shorter period moving average crosses the longer period moving average from below.
To generate short-term trading signals, generally, the 21-day moving average is considered a shorter period moving average, and the 50-day moving average is considered a longer-term moving average. For long-term trading, the 50-day moving average, 200-day moving average are considered as shorter and longer period moving averages.
In the daily chart of AAPL given below, the long term moving average crossover strategy is used to generate trading signals. On 2nd September 2016, a buy signal was generated when the 50-day moving average crossed above the 200-day moving average. The sell signal was generated on 20th December 2018 when the 50-day moving average crossed below the 200-day moving average.
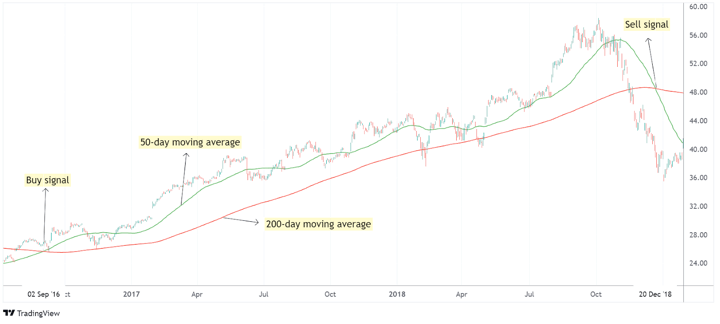
In quantitative analysis, cross-sectional and time-series momentum trading strategies come under trend trading strategies.
In cross-sectional momentum strategies, a long-short portfolio is created by studying the relative performance of the securities over a selected period.
For example, consider the S&P 500 index. To create a cross-sectional momentum strategy, we will calculate the performance of 500 stocks in the last 3 months. A long-short portfolio is created by taking a long position in the top 20% and a short position is taken in the bottom 20%. The portfolio will be rebalanced every 3 months.
Similarly, to create a time-series momentum strategy, we will create a long-short portfolio by considering the absolute performance of securities over a period of time. A cut-off will be defined and, according to the performance of the securities over a selected period, a long-short portfolio will be created.
For example, for the stocks in the S&P 500 index, a long position is taken in the stocks with more than 5% returns in the last 3 months and a short position is taken in stocks with less than -5% returns in the last 3 months.
In fundamental analysis, factor-based investing is an example of the trend trading approach. Factor-based investing selects the stocks to invest in by considering the factors that explain the stock returns.
These factors include the value, size, volatility, momentum, and growth. In addition to these, macroeconomic factors like inflation, interest rates, and Gross Domestic Product (GDP) are also considered.
Stocks selected based on these factors are expected to outperform the markets in the long term. The trends of these factors decide whether to buy or sell a stock.
Breakout Trading
In Breakout Trading, traders identify key levels of support and resistance and place trades when the price breaks through these levels, signalling a potential trend continuation.
For instance, if a stock has been consolidating within a specific price range and then surpasses a resistance level, it could trigger a buy signal.
Conversely, if the price falls below a support level, a sell signal may be generated. This strategy aims to capitalise on the momentum that often follows a significant price movement.
In the image below, breakout is shown where the price jumps to create a new resistance and support level. Since it was a rising support level, the buy signal was generated for maximising the returns in the future.
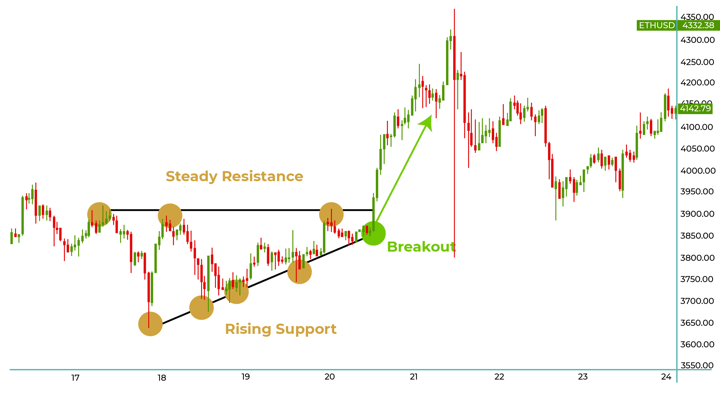
For seeing the clear buy signals and sell signals, you can refer to the image below:
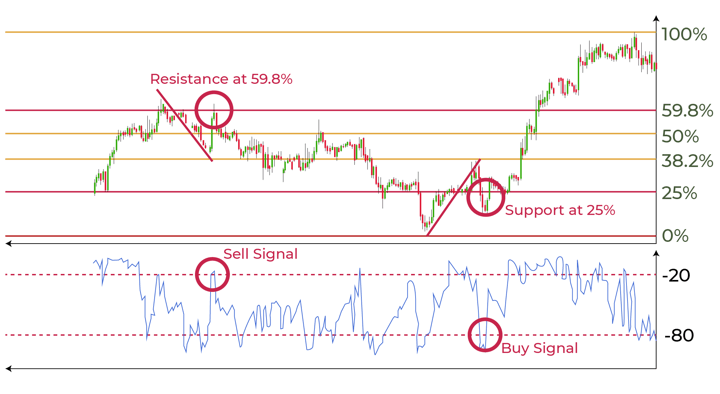
As you can see in the image above, there are a lot of support and resistance lines. The buy and sell signals get generated when there is a sudden change in the price to create a breakout. These breakouts are seen as “sell signals” and “buy signals”.
Trendline Trading involves drawing trendlines on a price chart to identify the direction of the prevailing trend. Traders look for opportunities to enter or exit positions when the price interacts with these trendlines.
For instance, the uptrend is when the price continually rises. In an uptrend scenario, the trader might initiate a buy when the price touches an ascending trendline.
Conversely, in a downtrend, when the price starts to fall, a sell signal could be triggered if the price breaches a descending trendline. Trendline trading helps traders visually interpret trend strength and potential reversal points.
In another scenario, which is a sideways trend, (also known as a horizontal or ranging market) the price of an asset moves within a relatively narrow range without making significant upward or downward progress. Traders often observe price oscillating between a defined level of support (where buying interest increases) and resistance (where selling interest increases). During a sideways trend, there may be frequent but relatively short-lived price fluctuations within this range, presenting challenges for trend-following strategies. Traders may employ range-bound or mean-reversion strategies to capitalise on price movements within the established range. (You can learn all about in this course on price action trading strategies.)
You can see these trends in the image below.
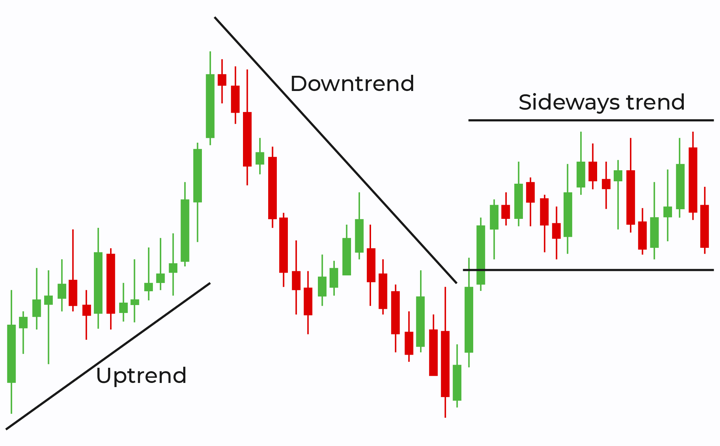
Parabolic SAR (Stop and Reverse)
The Parabolic SAR (Stop and Reverse) is a trend-following indicator that provides buy and sell signals based on price direction. When the dots of SAR are below the price, it suggests an uptrend, signalling a potential buy.
Conversely, when the dots of SAR are above the price, indicating a downtrend, it suggests a potential sell. This strategy helps traders ride the trend by providing dynamic stop-loss levels that adjust as the trend progresses.
Parabolic SAR can be seen in the image below.
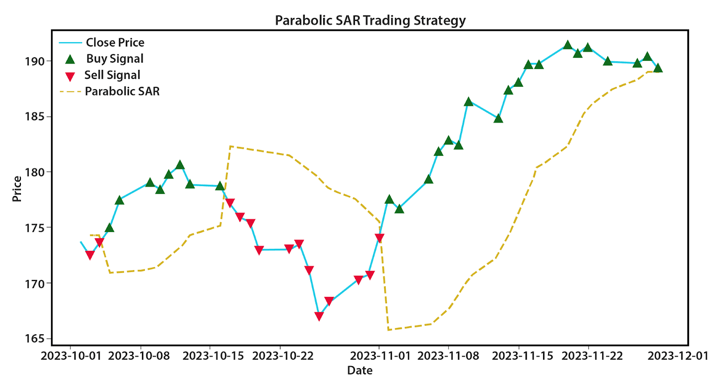
Mean-reverting strategies
The mean-reverting strategies aim to maximise returns from the temporary divergence of prices from their historical averages, with the expectation that prices will eventually revert to their mean values.
Let us see some mean-reverting strategies below.
In the Bollinger Bands Reversion strategy, traders anticipate that prices will revert to the mean, which is the middle band of the Bollinger Bands.
When the price deviates significantly from the mean (Moving Average) and reaches the upper or lower bands, it may be considered overbought or oversold.
Traders look for potential reversion opportunities, expecting the price to return towards the mean (Moving Average). This mean-reverting strategy helps identify potential turning points in the market.
You can see the image for Bollinger Bands trading below.
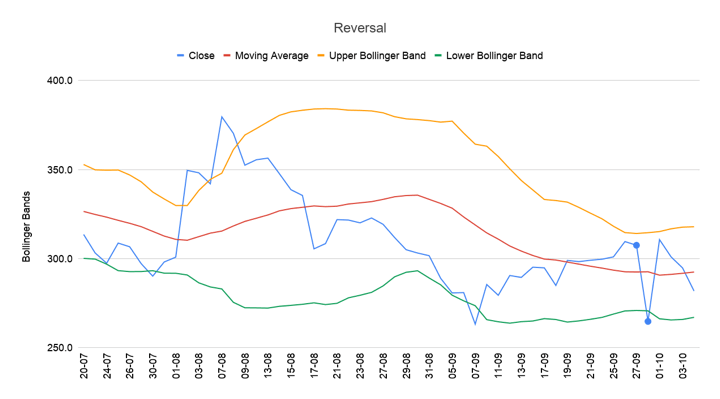
Pairs trading is a mean-reverting strategy that involves identifying two assets with a historical price relationship. Traders simultaneously take a long position in one asset and a short position in the other when the price spread between them deviates from its historical average. The expectation is that the spread will revert to its mean, providing an opportunity for maximising returns.
You can watch this video to learn more about pairs trading:
RSI (Relative Strength Index) Mean Reversion
The RSI Mean Reversion strategy utilises the Relative Strength Index, an oscillator that measures the magnitude of recent price changes, to identify overbought or oversold conditions. When the RSI indicates that an asset is overbought (above 70) or oversold (below 30), traders anticipate a mean reversion and potential buy or sell signals are generated. This strategy helps traders identify price levels at which an asset may revert to its historical average.
These overbought and oversold conditions can be seen in the image below.
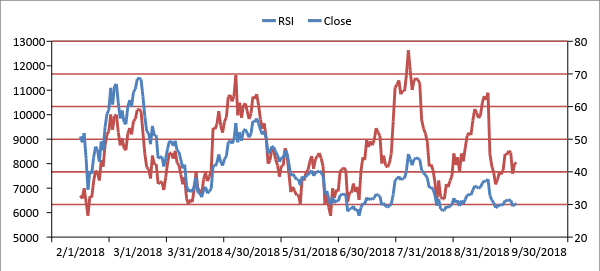
Statistical Arbitrage is a mean-reverting strategy that relies on statistical models to identify pricing inefficiencies between related assets. Traders create a portfolio by taking long and short positions in these assets based on historical relationships. As the assets deviate from their historical correlation, the strategy aims to capitalise on the expected mean reversion.
You can watch this video for a detailed explanation of the strategy:
Carry trade strategies
Now, let us find out the carry trade strategies.
Currency Carry Trade
The Currency Carry Trade is a popular strategy in the forex market. Traders borrow money in a currency with a low interest rate and use those funds to invest in a currency with a higher interest rate. The goal is to profit from the interest rate differential, known as the "carry."
This strategy assumes that the currency with the higher interest rate will appreciate, providing both interest income and potential capital gains.
Commodity Carry Trade
In the Commodity Carry Trade, traders focus on commodities with storage costs. They buy the physical commodity and simultaneously sell a futures contract for a later date. This strategy aims to capture the yield from the commodity's storage cost. For instance, in the oil market, if the storage cost is lower than the futures price, traders can profit from the carry by storing oil and selling futures contracts.
Bond Yield Carry Trade
The Bond Yield Carry Trade involves trading bonds to capture the yield spread between different bonds or fixed-income securities. Traders may borrow at a lower interest rate and invest in higher-yielding bonds, aiming to profit from the yield differential. This strategy relies on the expectation that the higher-yielding bonds will generate more income than the cost of borrowing.
Stock Dividend Carry Trade
In the Stock Dividend Carry Trade, traders focus on stocks with attractive dividend yields. They buy dividend-paying stocks and simultaneously short sell low-yielding or non-dividend-paying stocks. The goal is to profit from the dividend yield differential. Traders expect to earn more from the dividend income of the long position than the cost of funding the short position.
Carry trade strategies involve exploiting interest rate differentials, yield spreads, or income-generating opportunities across various financial instruments. Traders employing carry trade strategies seek to profit from the financing and investment differentials, taking advantage of favourable interest rate and yield conditions.
Suggested read: Carry Trade Strategy In Forex
Event-based trading strategies
Event-based strategies revolve around specific occurrences that have the potential to impact asset prices. Traders employing these strategies aim to capitalise on the price movements resulting from these events, leveraging their insights into market reactions and expectations.
Earnings Momentum Trading
Earnings Momentum Trading is an event-based strategy that focuses on trading around corporate earnings announcements. Traders analyse a company's earnings reports, specifically looking for surprises or deviations from market expectations. For example, if a company exceeds earnings forecasts, a trader might initiate a long position, anticipating positive momentum. Conversely, a disappointment in earnings might lead to a short position.
Economic Indicators Trading
Economic Indicators Trading involves reacting to significant economic releases, such as unemployment reports or GDP figures. Traders anticipate market movements based on the impact of these indicators on currency, commodity, or equity markets. For example, a positive employment report may lead to increased optimism about economic growth, influencing traders to go long on stocks.
Initial Public Offering (IPO) Trading
IPO Trading focuses on events surrounding the public debut of a company's stock. Traders analyse the prospectus, market sentiment, and demand for shares during the IPO. Depending on their assessment, traders may participate in the IPO to benefit from potential price appreciation during the initial trading days or short the stock if they anticipate a decline.
There is another one known as algorithmic trading strategies. Although the strategies are pretty much the same that we discussed above, you must know how to utilise the same in the algorithmic trading domain.
Take a brief walkthrough and learn about the types of algorithmic trading strategies in this insightful video that delves into the fascinating world of algorithmic trading strategies.
Suggested course: Technical Indicators Strategies in Python
Machine Learning based trading strategies
ML-based trading strategies are the contemporary practice. They are a mix of quantitative, technical and fundamental methods for each of the trading strategies mentioned above namely trending, mean reverting, break out, carry and event based.⁽²⁾
The three verticals of ML-based strategies are:
- Supervised learning trading strategies
- Unsupervised learning trading strategies
- Reinforcement learning trading strategies
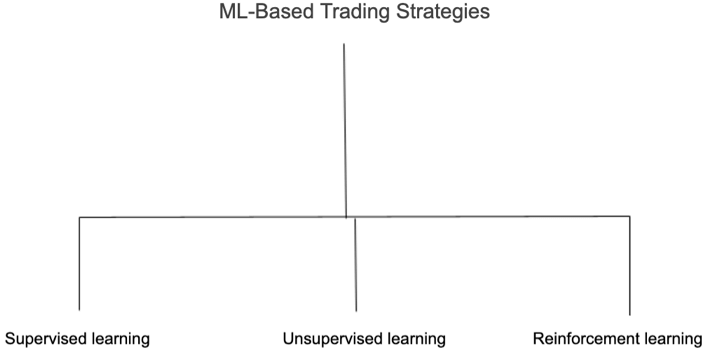
Supervised learning trading strategies
The supervised learning method for machine learning models consists of two main techniques, that is:
- Regression (for predicting the real numbers, of any variable such as stocks, commodities etc.)
- Classification (for classifying categories of a variable such as TSLA, GOOGL etc. in stocks)
Regression
Regression is a statistical process of determining relationships between variables. It helps one to understand how the value of the dependent variable changes when any one of the independent variables is varied.
It also allows comparing the effects of variables measured on different scales, such as the effect of price changes. In trading, regression is used extensively, especially in pairs trading strategy, and when it is required to evaluate the performance of a stock in comparison to market returns.
Linear regression
Linear Regression is one of the most widely known modelling techniques. Linear regression establishes a relationship between a dependent variable (Y) and one or more independent variables (X) using a best fit straight line.
If there is only one independent variable, then it is called a simple linear regression but if there are more than one independent variables, then it is called multiple linear regression.
Let us understand this with a diagram shown below:
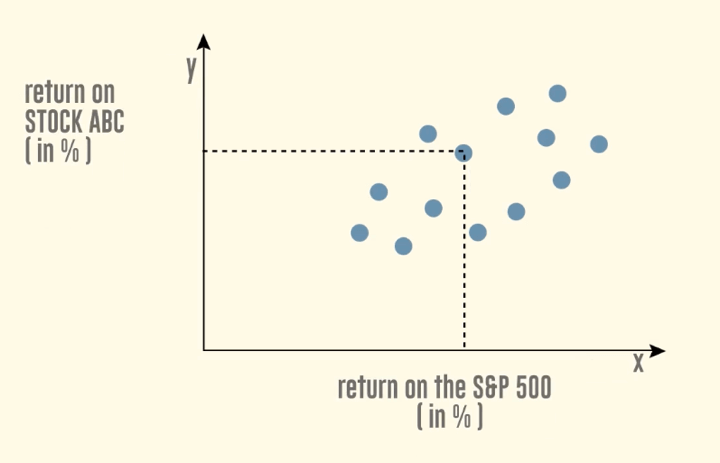
Linear regression
The above diagram shows the given data for “return on the S&P 500” on the x-axis and the predicted data for “return on stock ABC” on the y-axis. For calculating the regression line slope, you can use the Scikit-learn library in Python.
Scikit-learn library features algorithms for supervised and unsupervised models that include regression techniques, classification, clustering, random forest etc.
To calculate the y-intercept, which is the dependent/predicted variable, the formula goes as follows:
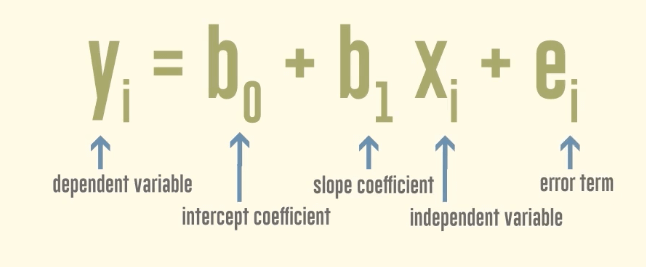
Formula for y intercept of linear regression
For example, if you calculated a slope of 1.5 and an intercept of 20, the final linear regression formula for the stock is:
y= 20 + 1.5x
Next in the supervised learning method is logistic regression.
Logistic regression
Logistic regression is similar to linear regression but with only one difference. The logistic regression model runs the result through a special non-linear function, the logistic function to produce the output “y”. Here, the output is binary or in the form of 0/1 or -1/1.
Logistic regression measures the relationship between the dependent variable and one or more independent variables by estimating probabilities using a logistic function.
Hence, the formula is as follows for logistic regression:
y = 1 / 1+ e-x
Classification
Classification is one of the methods which is applied using a Support Vector Classifier (SVC) technique and is part of an unsupervised learning method in machine learning. The classification technique maps the input into a discrete class or a category as shown in the image below:
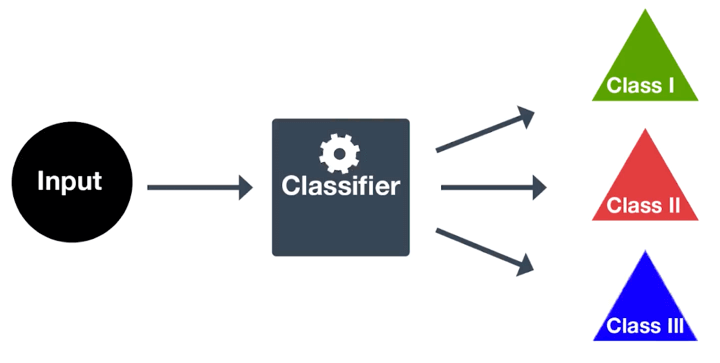
Classification
For instance, the categories in the trading domain can be classified as entry position and exit position in any of the following markets - a stock, commodity, bond, or derivative.
Recommended read: Machine Learning Classification Strategy in Python
Random forest
Random Forest, also called Random Decision Forests, is a method in machine learning capable of performing both regression and classification tasks. It is a type of ensemble learning that uses multiple learning algorithms for prediction.
Random Forest comprises decision trees, which are graphs of decisions representing their course of action or statistical probability. These multiple trees are plotted to a single tree called the Classification and Regression (CART) Model.
To classify an object based on its attributes, each tree gives a classification that is said to vote for that class. The forest then chooses the classification with the maximum number of votes. For regression, it considers the average of the outputs for different trees.
Working
- Let us assume the number of cases as “N”. Then, randomly but with replacement, the sample of these N cases is taken out, which will be the training set.
- Considering M to be the input variable, a number m is selected such that m < M. The best split between m and M is used to split the node. The value of m is held constant as the trees are grown.
- Each tree is grown as large as possible.
- By aggregating the predictions of n trees (i.e., majority votes for classification, the average for regression), random forest predicts the new data.
For instance, in the stock market, random forest is used to identify a stock’s behaviour (taking the past performance of the stock in ‘n’ number of years) in terms of expected returns.
Unsupervised learning trading strategies
Unsupervised learning is a type of machine learning in which only the input data is provided and the output data (labelling) is absent. Algorithms in unsupervised learning are left without any assistance to find results and in this method of learning, there are no correct or wrong answers.
K-Means clustering
K-Means Clustering is a type of unsupervised machine learning that groups data based on similarities. K-Means is one technique for finding subgroups within datasets. One difference in K-Means versus that of other clustering methods is that in K-Means, we have a predetermined amount of clusters while other techniques do not require that we predefine the number of clusters.
The algorithm begins by randomly assigning each data point to a specific cluster with no one data point being in any two clusters. It then calculates the centroid, or mean of these points.
The object of the algorithm is to reduce the total within-cluster variation. In other words, we want to place each point into a specific cluster, measure the distances from the centroid of that cluster and then take the squared sum of these to get the total within-cluster variation. Our goal is to reduce this value.
The process of assigning data points and calculating the squared distances is continued until there are no more changes in the components of the clusters, or in other words, we have optimally reduced the in-cluster variation.
Recommended read: K-Means Clustering Algorithm For Pair Selection In Python
Reinforcement learning trading strategies
Reinforcement learning is a way to encourage or change a particular unwanted behaviour by the system. Whenever the system gets a reward for giving the desired result (as fed to the system), it is positively reinforced and when the system does the opposite of the desired result, it is negatively reinforced. This way the machine learning system learns.
It is very much similar to how human beings learn. When they get the desired result in a field, say, a good score in an examination, they are rewarded with a good job. Here is a diagrammatic representation of how a machine learning model works with reinforcement learning:
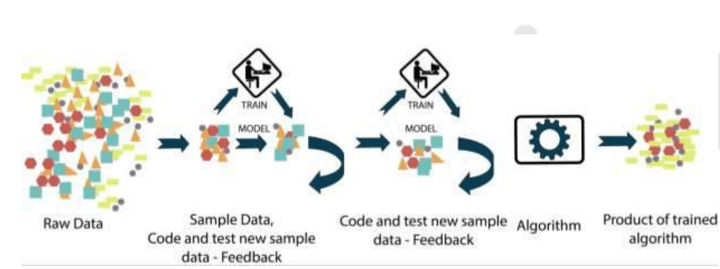
Recommended course: Introduction to machine learning for Trading
Machine learning trading strategies are best applied with the help of the popular computer language Python. There is a Python package known as Scikit-learn, which is developed specifically for machine learning and features various classification, regression and clustering algorithms.
Delve into the fundamentals, the importance of learning machine learning, various algorithms, real-world applications in trading, insights into machine learning-based trading systems, live trading signal demonstrations, and guidance on evaluating and implementing these strategies.
Methods of creating trading strategies
There are three main methods used to design a trading strategy:
- Technical analysis
- Fundamental analysis
- Quantitative analysis
- Machine learning for designing trading strategies
Technical Analysis
Involving the examination of price charts and patterns, technical analysis is a method that identifies trading opportunities by scrutinising trends, and volumes, and predicting future price movements. For instance, a technical analyst might use moving averages or support and resistance levels to make buy or sell decisions based on historical price data.
Fundamental Analysis
Crucial for determining intrinsic stock value, fundamental analysis delves into industry, economic factors, and company fundamentals. For instance, an investor using fundamental analysis might assess a company's earnings, revenue growth, and financial health to gauge its overall worth before making investment decisions.
Quantitative Analysis
Employing mathematical models and statistical techniques, quantitative analysis predicts stock prices by assessing historical and current data. Quants might use algorithms and statistical models to identify patterns and trends, such as employing quantitative strategies based on statistical arbitrage or mean-reversion principles.
Machine Learning for Designing Trading Strategies
Leveraging contemporary machine learning practices, this involves using artificial intelligence to automatically detect patterns and execute trades. For instance, machine learning algorithms can analyse vast datasets, learn from historical market behaviour, and autonomously adapt to evolving market conditions, optimising trading strategies over time.
These 15 most popular strategies are explained in this video below.
Portfolio of trading strategies
The strategies explained in this article can be combined to create a portfolio. For example, you can combine the strategies under fundamental analysis and technical analysis. Fundamental analysis will be used to select the asset to trade and technical analysis will be used to time the entry.
Now, the market moves in different regimes. So, a momentum strategy may not work properly when the market is not trending. At the same time, a mean reversion strategy may not perform the best when the market is trending. Hence, you can combine momentum with mean reversion strategies to generate consistent returns.
Here, you can allocate some of the capital to momentum strategies and some to mean reversion strategies (based on the expected market situation). Similarly, you can allocate the capital to different strategies depending on the situation such as market volatility (current and expected).
Also, you can trade in a variety of tradeable items such as Forex (currency), equity shares, commodities etc. to create a portfolio and apply different strategies as mentioned above.
Moreover, the strategies namely, trend trading strategies, mean-reverting strategies, break-out, carry and event-based can be modelled with the help of machine learning models/methods/strategies as described above.
Here, combining the strategies will:
- Decrease the overall maximum drawdown
- Increase the stability of returns, and
- Diversify the assets that we trade
Be sure to check out this power-packed webinar by speaker Prodipta Ghosh (Vice President, QuantInsti), sharing his expert insights on Quantitative Portfolio Management Strategies.
Moreover, learn the difference between buying stocks and creating a winning portfolio in this video below.
Innovations in trading strategies
Trading strategies have come a long way from the days of gut feelings and hand-drawn charts. The years leading up to the present have witnessed a whirlwind of innovation, transforming the way we trade. Here's a glimpse into some key advancements:
Technological Advancements:
- The rise of electronic trading: This shift from floor-based trading to electronic platforms, starting in the 1970s, opened the door for faster execution, lower costs, and access to wider markets.
- Algorithmic trading: The introduction of computers and algorithms in the 1980s allowed for automated trade execution based on predefined rules and mathematical models. This led to high-frequency trading (HFT) and other sophisticated strategies.
- Data explosion and analytics: The vast amount of financial data available today, coupled with powerful analytical tools, enables traders to identify complex patterns, assess risks, and optimise their strategies more effectively.
- Mobile trading: Mobile apps have made trading accessible anywhere, anytime, democratising participation in the market and catering to new generations of investors.
Strategic innovations:
- Quantitative trading: This approach relies on statistical analysis and mathematical models to identify market trends and generate trading signals. It led to the development of complex strategies like statistical arbitrage and mean reversion.
- High-frequency trading (HFT): Using algorithms and high-speed connections, HFT strategies exploit tiny price discrepancies in milliseconds, resulting in rapid execution and large volumes.
- Machine learning and AI: AI algorithms are increasingly used to analyze data, identify patterns, and even forecast market movements. This is paving the way for new and potentially predictive trading strategies.
- Social trading and copy trading: Platforms allow individuals to follow and copy the trades of successful traders, democratising access to expert strategies and insights.
Beyond traditional markets:
- Cryptocurrency trading: The emergence of Bitcoin and other cryptocurrencies has led to new, highly volatile markets and unique trading strategies, often using technical analysis and sentiment indicators.
- Decentralised finance (DeFi): This emerging ecosystem offers alternative investment avenues like lending, borrowing, and derivatives trading, requiring specialised strategies and risk management approaches.
Recent Developments:
While the year is still young, some key trends include:
- Focus on ESG investing: Environmental, social, and governance (ESG) factors are gaining importance in trading strategies, with investors seeking ethical and sustainable returns.
- Rise of Fintech: Fintech startups are disrupting traditional finance, offering innovative trading platforms, robo-advisors, and new trading instruments.
- Regulatory evolution: Regulatory landscapes are adapting to evolving markets and technologies, impacting trading strategies and compliance requirements.
Overall, the landscape of trading strategies has undergone a radical transformation thanks to continuous innovation. Technology, data, and new asset classes have opened up exciting possibilities, while also demanding continuous learning and adaptation from traders. The future promises even more advancements as AI, blockchain, and other technologies continue to integrate with the financial world.
Common mistakes to avoid in different trading strategies
While specific mistakes can vary depending on the chosen trading strategy, some general pitfalls apply across the board. Here are some common mistakes to avoid in different trading strategies:
General mistakes
- Emotional trading: Letting fear, greed, or revenge influence your decisions. Stick to your trading plan and remain objective.
- Poor money management: Risking too much in a single trade, not using stop-loss orders, or ignoring risk parameters.
- Overconfidence: Riding winning streaks or failing to acknowledge losses can lead to reckless decisions. Practice humility and continuous learning.
- Lack of research and analysis: Rushing into trades without a proper understanding of the market, fundamental factors, or technical indicators.
- Chasing returns: Obsessing over short-term gains can lead to bad trades and overlook long-term opportunities.
Mistakes specific to some strategies
- Day trading: Overtrading, neglecting news and fundamental analysis, focusing on low-liquidity stocks, or ignoring market trends.
- Swing trading: Holding onto losing positions for too long, failing to adapt to changing market conditions, or neglecting timeframe consistency.
- Position trading: Not considering long-term fundamentals, reacting to short-term noise, or neglecting portfolio diversification.
- Algorithmic trading: Overfitting the model to historical data, failing to backtest in different market conditions, or ignoring real-time market anomalies.
- High-frequency trading: Not considering latency and infrastructure costs, neglecting regulatory changes, or focusing solely on market microstructure exploits.
How to choose the right trading strategy?
Now let us see the tips so that you can avoid the mistakes mentioned above and choose the right trading strategy.
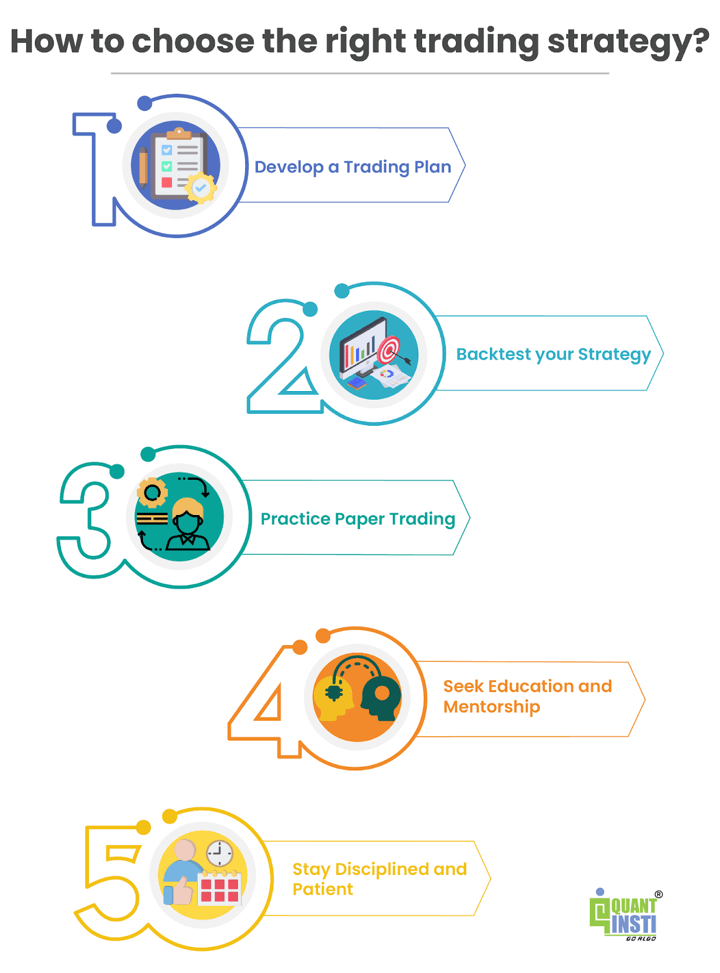
- Develop a trading plan: Outline your strategy, risk management rules, and entry/exit criteria.
- Backtest your strategy: Test your strategies with historical data to assess their effectiveness.
- Practice paper trading: Simulate real-world trading without risking capital.
- Seek education and mentorship: Learn from experienced traders and continuously improve your skills.
- Stay disciplined and patient: Successful trading requires discipline, patience, and the ability to stick to your plan.
Conclusion
Traders equipped with a comprehensive toolkit of strategies are better positioned to make informed decisions, effectively manage risks, and optimise outcomes. As this exploration concludes, it highlights the significance of continuous learning and strategic refinement in the ever-evolving world of trading.
Armed with this knowledge, investors can navigate financial markets with confidence, leveraging the versatility of trading strategies to thrive in a landscape characterised by complexity and constant change.
If you are starting your journey in quantitative trading, you might want to learn basic technical trading strategies, like the trend-based strategy and the Bollinger bands strategy, as well as other strategies. You can check out our course on Quantitative Trading Strategies and Models which can help you learn all of this as well as trade these strategies in the live markets! So, go ahead and enrol in the course for a great trading experience!
Authors: Chainika Thakar and Varun Pothula
Note: The original post has been revamped on 20th February 2024, for the accuracy and recentness.
Disclaimer: All data and information provided in this article are for informational purposes only. QuantInsti® makes no representations as to accuracy, completeness, currentness, suitability, or validity of any information in this article and will not be liable for any errors, omissions, or delays in this information or any losses, injuries, or damages arising from its display or use. All information is provided on an as-is basis.